Streetlight effect and forecasting
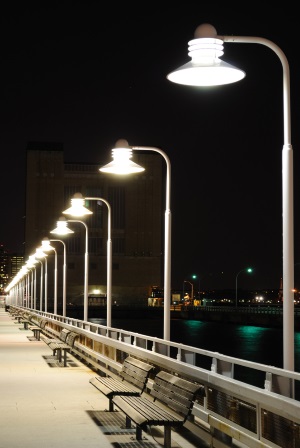
A policeman sees a drunk man searching for something under a streetlight and asks what the drunk has lost. He says he lost his keys and they both look under the streetlight together. After a few minutes the policeman asks if he is sure he lost them here, and the drunk replies, no, and that he lost them in the park. The policeman asks why he is searching here, and the drunk replies, “this is where the light is.” David H. Freedman (2010). Wrong: Why Experts Keep Failing Us.
One of the most paradoxical things about “classic” forecasts is that they look for the average – sometimes median – value of the future demand, while this average case, as we will see below, is mostly irrelevant. Whenever daily, weekly or monthly forecasts are being used, these can be considered as average forecasts. Why? Because, other kinds of forecasts, like quantile forecasts, are not additive which makes them fairly counter-intuitive. In fact, most supply chain practitioners aren’t even aware that alternatives to “classic” forecasts exist in the first place.
However, business-wise, as far as inventory is concerned, it’s not the middle ground that costs money, rather it’s the extremes. On the one hand, there is the unexpectedly high demand that causes a stock-out. On the other hand, there is the unexpectedly low demand that causes dead inventory. When the demand level is roughly where it was expected to be, inventory levels gently fluctuate, and inventory rotates very satisfyingly.
As a result, there is no point in optimizing the average case, i.e. when inventory is rotating very satisfyingly, because there is little or nothing to improve in the first place. It’s the extremes that need to be taken care of. Actually, most practitioners are keenly aware of this issue, as their top 2 problems are to improve the service quality on the one hand (i.e. mitigating the unexpectedly high demand), while keeping the stock levels in check on the other hand (i.e. mitigating the unexpectedly low demand).
Yet, since we have agreed that supply chain challenges are mainly concerned with the “extremes”, why do many companies still look for answers through “average” forecasts? I believe that supply chain management, as an industry, is suffering from a bad case of drunkard’s search, a problem called the streetlight effect. Classical tools and processes are shedding light on “average” situations which barely need to be enlightened any further, while leaving entirely in the dark whatever lies at the extremes.
A frequent misconception consists of thinking that improving the “middle” case should also marginally improve the extremes. Alas, statistical forecasting is counter-intuitive and basic numerical analysis shows that this is simply not the case. Statistical forecasting is like a microscope: while being incredibly sharp, it’s focus is also incredibly narrow.
Trying to fix your supply chain problems through classic “average” forecasts is like trying to diagnose what’s wrong with your car which is refusing to start by putting every single car part under a microscope starting with the engine. At this rate, you will probably never manage to diagnose that your car won’t move because there is no more gas, which in hindsight, was a pretty obvious problem.
However, this is not the end of the insanity. Now imagine that the repair guy, after failing to diagnose why your car isn’t moving, started to claim that his diagnosis had failed because his microscope didn’t have enough resolution. And now the repair guy is asking you for more money so that he can buy a better microscope.
Well, a similar scenario is presently happening in many companies: the previous forecasting initiative has failed to deliver the desired inventory performance, and companies double down with another forecasting initiative along the very lines that caused the first initiative to fail in the first place.
At Lokad, it took us 5 years to realize that the classic forecasting approach wasn’t working, and even worse, that it will never be working no matter how much technology we would add to the case, just like switching to a $27M ultra-high resolution microscope would never have helped the repair guy to diagnose your empty tank. In 2012, we uncovered quantile forecasts that we steadily kept improving; and suddenly, things started working.
Those five years of steady ongoing failures felt long, very long. In our defense, when an entire industry works on false promises which can be traced back to university manuals, it’s not that easy to start thinking outside the box when the box itself is so huge that you can spend your life wandering inside in circles and never hitting the walls.
Reader Comments (1)
Very nice analogy, thanks Joannes Vermorel. A year ago | Victor