Deep Learning as 5th gen forecasting engine
As part of our core commitment to deliver the most accurate forecasts that technology can produce, we are proud to announce that our 5th generation of forecasting engine is now live at Lokad. This engine is bringing the largest accuracy improvement that we have ever managed to achieve in a single release. The engine’s design relies on a relatively recent flavor of machine learning named deep learning. For supply chains, large forecasting accuracy improvements can translate to equally large returns, serving more clients, serving them faster, while facing less inventory risks.
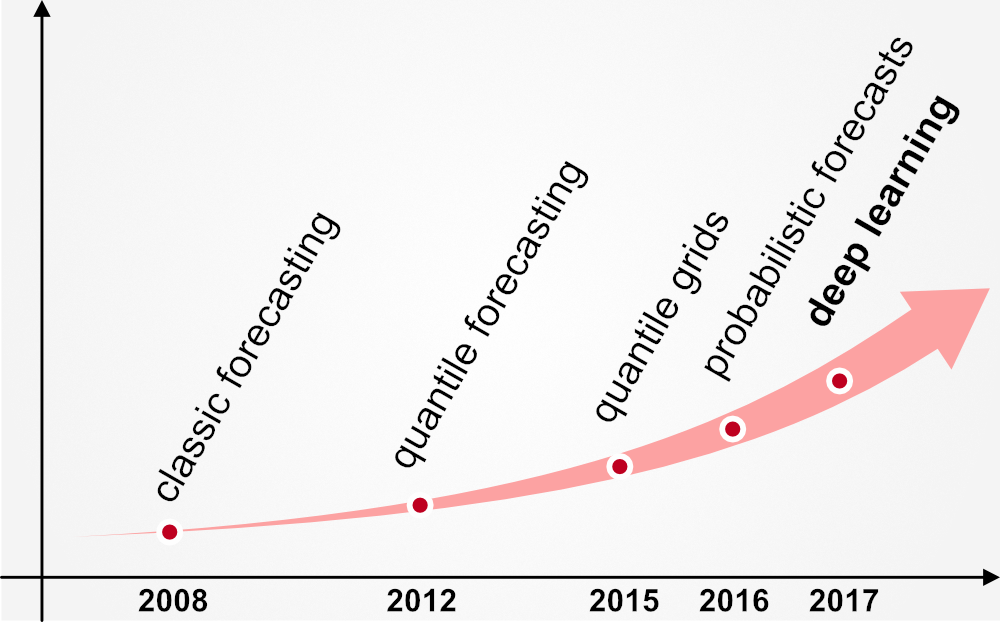
From probabilistic forecasting to deep learning
About 18 months ago, we were announcing the 4th generation of our forecasting technology. The 4th gen was the first to deliver true probabilistic forecasts. Probabilistic forecasts are essential in supply chains because the costs are concentrated on the statistical extremes, when demand happens to be unexpectedly high or low. In contrast, traditional forecasting methods - like traditional daily, weekly or monthly forecasts - that only focus on delivering median or average forecasts, are blind to the problem. As a consequence, those methods usually fail to deliver satisfying returns for companies.
Partly by chance, it turns out that deep learning happens to be heavily geared toward probabilistic forecasts by design. The motivation for this perspective was, however, entirely unrelated to supply chain concerns. Deep learning algorithms are favoring optimization built on top of a probabilistic / Bayesian perspective with metrics like cross entropy because these metrics provide huge gradient values that are especially suitable for the stochastic gradient descent, the “one” algorithm that makes deep learning possible.
In the specific case of supply chains, it happens that the foundations of deep learning are fully aligned with the actual business requirements!
Beyond the hype of artificial intelligence
Artificial intelligence - powered by deep learning in practice - has been the buzzword of the year in 2017. Claims are bold, enthralling and, well, fuzzy. From Lokad’s vantage point, we observe that the majority of these enterprise AI techs are not living up to their expectations. Very few companies can secure over half a billion USD in funding, like Instacart, to gather a world-class deep learning team in order to successfully tackle a supply chain challenge.
With this release, Lokad is making AI-grade forecasting technology accessible to any reasonably “digitalized” company. Obviously, the whole thing is still powered by historical supply chain data, so the data must be accessible to Lokad; but our technology requires zero deep learning expertise. Unlike virtually every single “enterprise” AI techs, Lokad does not rely on manual feature engineering. As far as our clients are concerned, the upgrade from our previous probabilistic forecasts to deep learning will be seamless.
Lokad is the first software company to provide a turnkey AI-grade forecasting technology, accessible both to tiny 1-man ecommerces and yet scaling up to the largest supply chain networks that can include thousands of locations, and a million product references.
The age of GPU computing
Deep learning remained somewhat niche until the community managed to upgrade its own software building block to take advantage of GPUs (graphic processing units). Those GPUs differ largely from CPUs (central processing units), which are still powering the vast majority of apps nowadays with the notable exceptions of computer games, which are intensively relying on both CPUs and GPUs.
Along with the complete rewrite of our forecasting engine for this 5th iteration, we have also significantly upgraded the low level infrastructure of Lokad. Indeed, in order to serve companies, the Lokad platform now leverages GPUs as well as CPUs. Lokad is now taking advantage of the GPU-powered machines that can be rented on Microsoft Azure, the cloud computing platform that supports Lokad.
Through the massive processing power of the GPUs, we are not only making our forecasts more accurate, we are making them much faster too. Through a grid of GPUs, we are now typically getting the forecasts about 3x to 6x faster, for any sizeable datasets (*).
(*) For ultra-small datasets, our 5th gen forecasting engine is actually slower, and takes a few more minutes - which is largely inconsequential in practice.
Product launches and promotions
Our 5th generation forecasting engine is bringing substantial improvements to hard forecasting situations, most notably product launches and promotions. From our perspective product launches, albeit very difficult, remain a tad easier than promotion forecasts. The difference in difficulty is driven by the quality of the historical data, which is invariably lower for promotions compared to product launches. Promotion data gets better over time once the proper quality assurance processes are in place.
In particular, we are seeing deep learning as a massive opportunity for fashion brands who are struggling with product launches that dominate their sales: launching a new product isn’t the exception, it’s the rule. Then, as color and size variants vastly inflate the number of SKUs, the situation is made even more complex.
Early access to deep forecasting
We are planning to gradually upgrade our entire clientbase to our newest forecasting engine. This gradual deployment is intended to make sure we don’t inadvertently introduce regressions where the latest version could happen to be less accurate than the older one. As the version 5.0 is externally identical to the version 4.0, the upgrade will be fully transparent. Clients will only notice the extra accuracy. By the end of Q1 2018, all the forecasts generated through Envision will be powered by 5.0.