Predictive Optimization For Fashion
Fashion is driven by novelty. A fashion brand needs to design and push the right product at the right time, at the right price and with the stock quantity that happens to be just enough to serve the market. Then, promotions are used first to amplify the demand and second to liquidate any remaining excess of stocks. Lokad provides a statistical software solution that delivers predictive optimization at every step of the product life cycle, from inception to liquidation. Our technology embraces exactly what makes fashion so incredibly challenging: ubiquitous cannibalizations and substitutions, erratic trends and seasonalities, never-ending streams of new products, highly dynamic prices, etc.
Ed. The following content does not apply to hard luxury, which follows a very different set of rules and constraints. For more information, see Predictive Optimization for Hard Luxury.
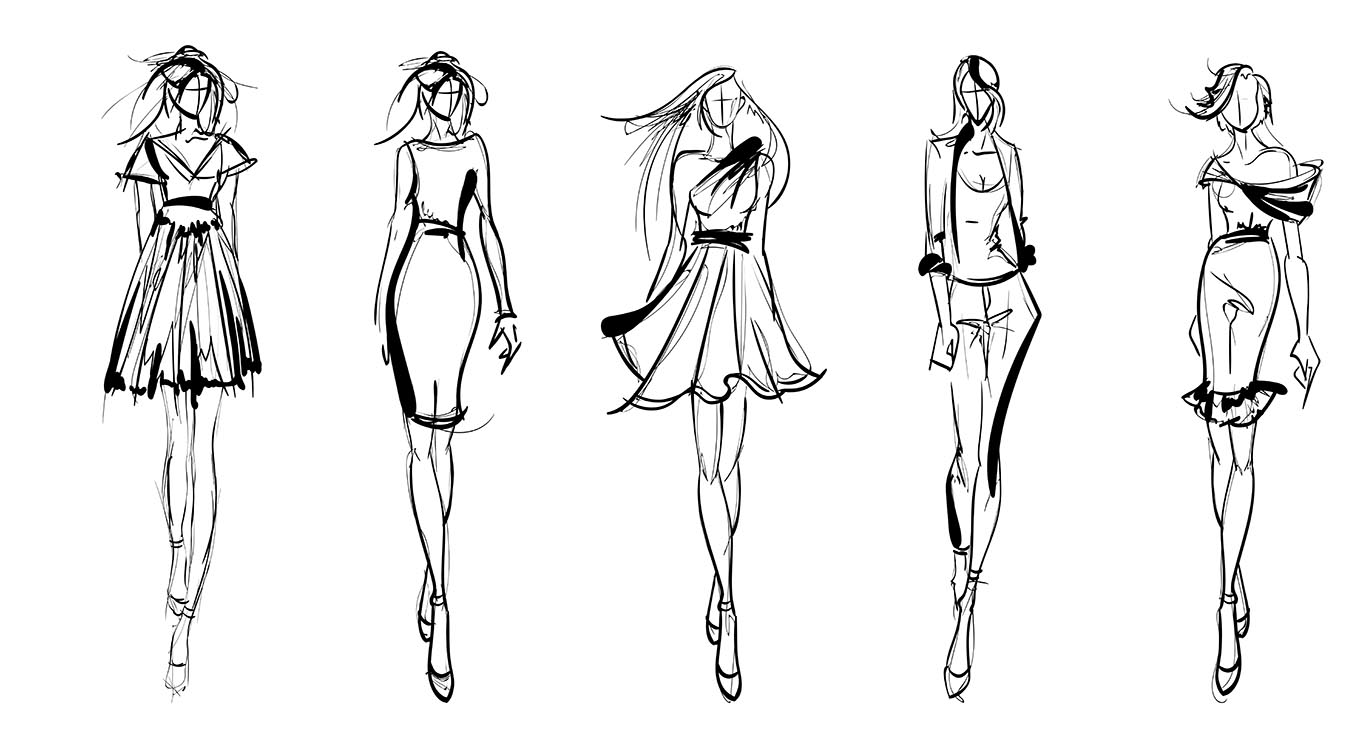
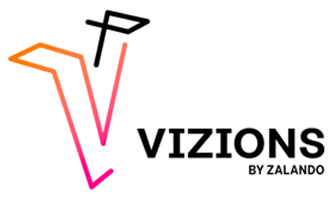
Vizions by Zalando: Europe's first platform conference April 20th, 2017 Berlin
Let's start with an innocent question. Why do you think there are Sales ? Nowadays, Winter Sales, Summer Sales, and a number of smaller events in between are an institution, especially in fashion. (...) But why do they exist in the first place ? They exist to allow retailers to get rid of overstock. In the first place, Sales are a manifestation of a forecast gone wrong. It can be argued that today they are much more than that. True, but still the initial goal remains.
Joannes Vermorel, Founder of Lokad

LOKAD TV
Take a look at our interviews on Lokad TV and learn more about Supply Chain and fashion.
End-to-end optimization over the full product life-cycle
Phase 1 - pre-collection
Many fashion brands are launching thousands of new references per collection - considering sizes, colors, point of sales, etc.
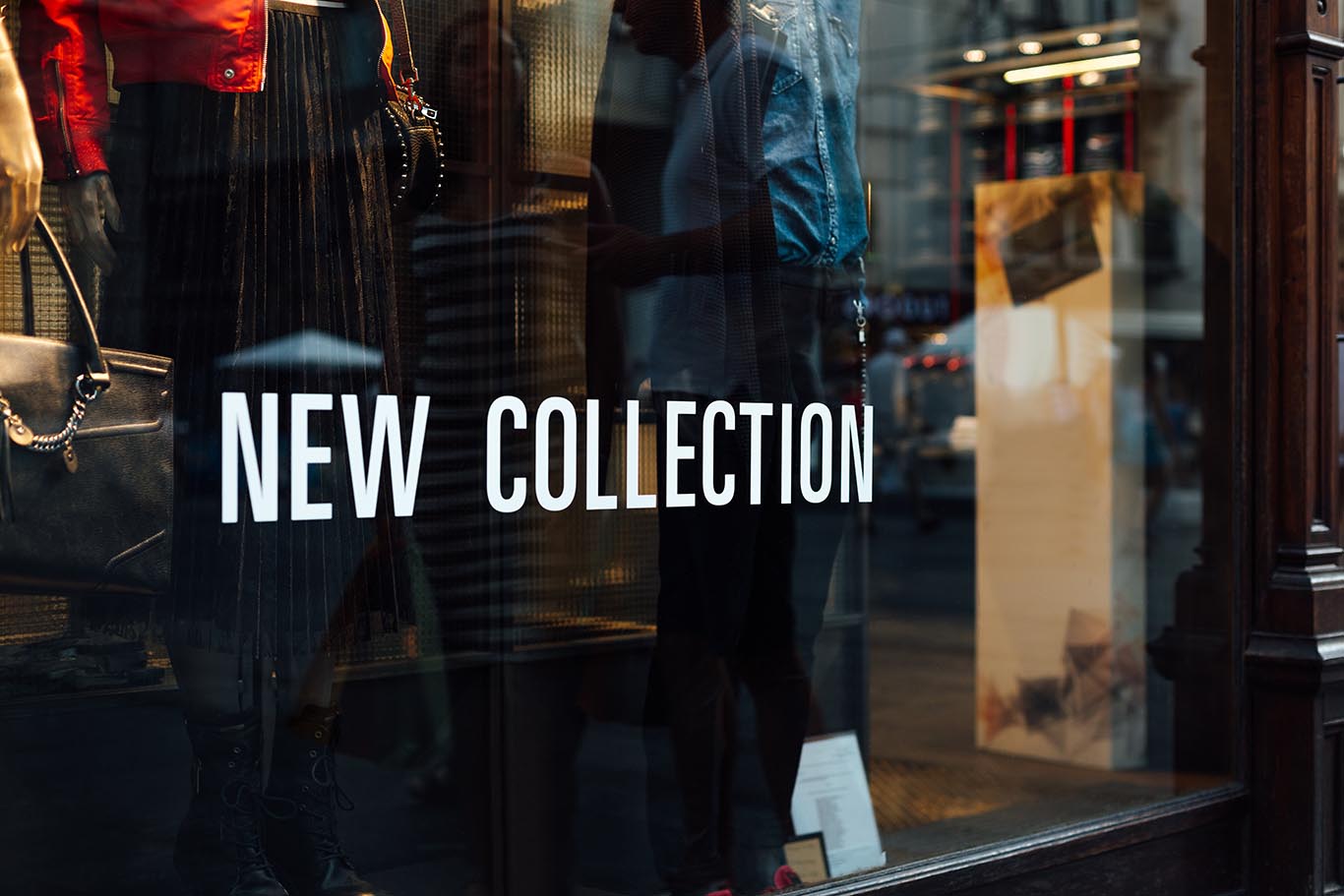
Over the last decade, the most successful brands have demonstrated their capacity to adhere ever more to the latest trends, increasing their number of collections per year and reducing their lead times.
Lokad’s predictive optimization technology tackles every single decision through the entire life-cycle for every product from the brand. Our technology is a mix of machine learning - used to extract patterns or predictions from the data - and of numerical optimization - used to generate optimized decisions.
The preparation of the new collection starts with the delivery of an assortment optimization - also referred to as a range plan. The Lokad technology lets you compose the right range of sizes, colors and shapes on top of the initial designs. This range plan is intended to fit as closely as possible to the wishes and expectations of your customer base. Lokad can help you generate the business case that supports the range plan, and offers the possibility to planners to refine the plan with their high-level insights - as opposed to micro-managing every single product variant.
As soon as the range plan is finalized, we deliver a purchasing optimization, suggesting exactly how many units should be produced or purchased for every single product variant - and when to order as well. Naturally, most products have never been sold before. This task is typically complicated by multiple MOQ (Minimal Order Quantities) constraints, price breaks, as well as varying lead times (e.g. Chinese New Year slowing down production in Asia). Lokad can also optimize the transportation mix (e.g. sea vs. air freight) and the source mix (e.g. overseas suppliers vs local suppliers).
As an example, our MOQ solver can tackle multiple overlapping MOQ constraints: there might be a MOQ at the product level (e.g. a minimum of 100 units per product for every purchase order), another MOQ at the fabric level (e.g. a minimum of 3000 meters of fabric per color), and a final MOQ at the supplier level (e.g. a minimum of $50,000 worth of merchandise purchased per order). Addressing all of these MOQs while keeping stock levels under control is a major hassle when MOQs are processed manually. Lokad streamlines the process entirely through numerical solvers that allow to identify the most profitable purchase order “enveloppe” that satisfies all given constraints.
Phase 2 - post collection launch
When the collection launch looms ahead, we deliver a stock allocation optimization, deciding exactly how many units to allocate to each distribution center and/or to each store. In store, the stock has two purposes: not only servicing the demand, but also attracting the clientele in the store. The allocation optimization properly takes into account this merchandizing angle, making the most of every store. The optimization takes into account storage capacity limits (e.g. shelf space), as well as the limited capacity of the ground teams to cope with large incoming batches of products. Bundles and lots - which are handy to lower manutention costs - are also taken care of.
In order to stick to the latest trends, we identify best-sellers and slow-movers. The identification of the best-sellers, intended to trigger early replenishments, can be done with limited stock quantities, only probing the market in a limited number of stores - possibly only probing the market through the e-commerce platform. Conversely, the early identification of slow-movers is also important in order to remove as soon as possible those products from the shelves that they occupy at the expense of better products.
For most fast fashion brands, discounts and other promotional mechanisms are part of the brand’s DNA. Lokad delivers pricing optimization capabilities, suggesting when to lower the price in order to ensure that there won’t be any left over at the end of the collection, while maximizing the overall amount of gross margin
We also take into account discounts originating from loyalty programs - uniformly applied over many products - which may replace and sometimes supplement discounts at the product level.
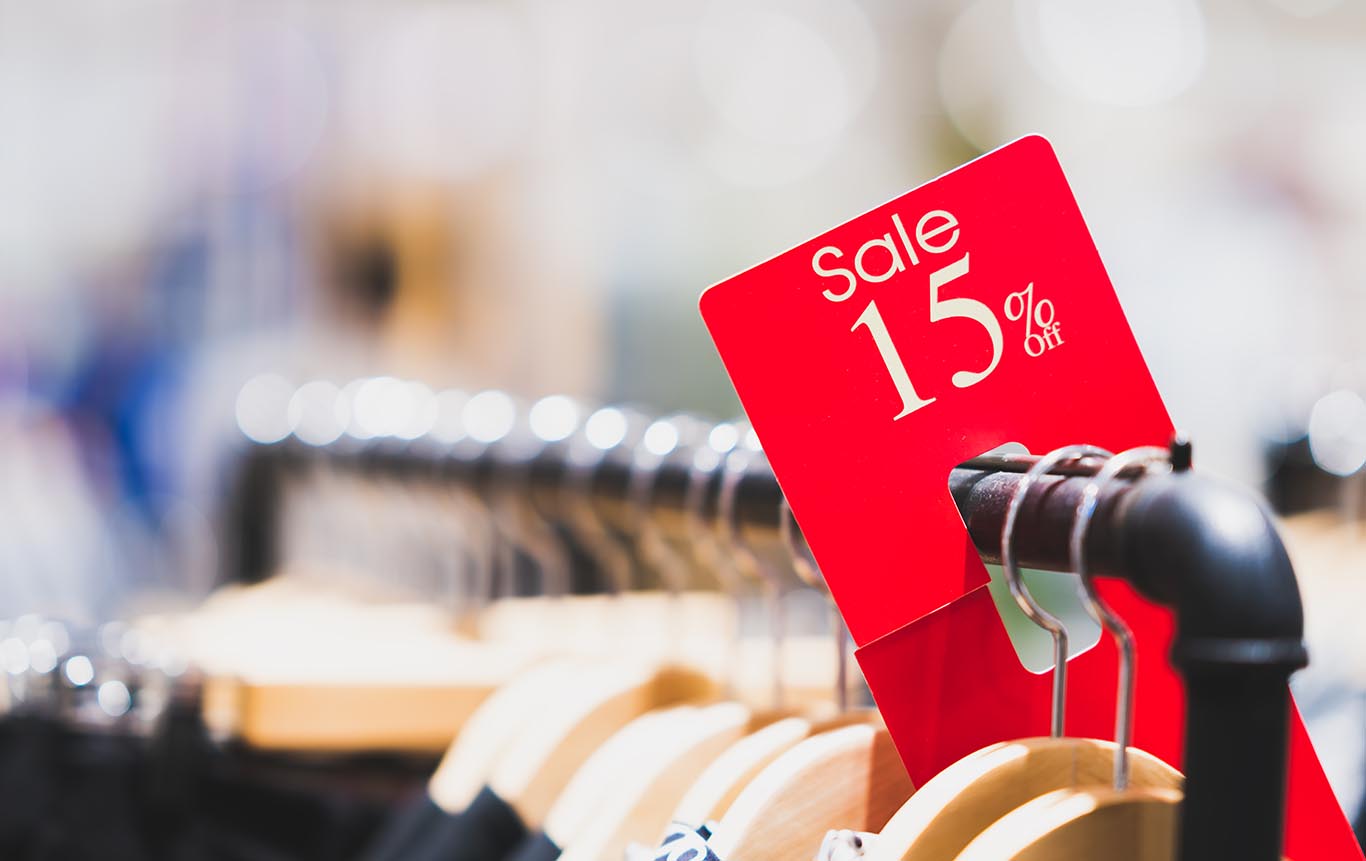
Assortment optimization, purchasing optimization, stock allocation optimization, identification of best-sellers and slow-movers, pricing optimization: Lokad can be used to optimize all these decisions - and more - with a consistent end-to-end perspective on the products’ full lifecycle. However it’s also possible to start small with a more narrow scope. For any inquiries, drop us an email at contact@lokad.com
Fashion requires non-classic demand forecasts
The optimization of decisions involving stocks and prices requires accurate insights about the future. However, the classic demand forecasting perspective is mostly dysfunctional as far as fashion is concerned. Lokad has therefore developed unique forecasting capabilities engineered at their core around the challenges faced by fashion companies.
We work with probabilistic forecasts: we consider all the possible futures, and we compute their respective probabilities. Indeed, the demand uncertainty is irreducible in fashion. There is no hope of producing a “perfect” forecast. Traditional methods expect decision to be taken against the “One” forecast, making those decisions fragile against forecast errors.
Instead of dismissing uncertainty, we embrace it. Through probabilistic forecasts, we balance risks and opportunities.
The forecasts operate at the assortment level in order to take into account the cannibalization and substitution effects. Forecasting the demand of a product in isolation is meaningless, as the demand for this product is strongly influenced by the presence - or absence - of similar products that all compete for the same customers. Traditional methods focusing on time-series forecasts entirely miss this point, and the situation usually gets worse when safety stocks or service levels are also used.
The demand is conditioned by the pricing, which is an integral part of our forecast. Not only is the future demand a matter of probabilities, but those probabilities are influenced by the price of the product - an actionable lever at the disposal of the company.
Once again, assortment matters, and the uplift of demand depends on the overall promotional intensity. It’s unwise to expect the same uplift if the product is the only one being promoted in the store, compared to a promotion applied to the whole store. Forecasts don’t just stop with the demand; returns and lead times must be forecasted as well.
Every source of uncertainty requires proper statistical forecasts, and while future demand is central, there are needs beyond demand forecasting and we have engineered our technology accordingly. Those forecasts are also usually probabilistic and tend to be combined with the demand forecasts themselves - e.g. forecasting the demand over the lead time. The latest generation of our forecasting technology relies on differentiable programming. This descendent of deep learning is particularly suitable to deal with sparse and intermittent demand as commonly observed in the fashion industry.
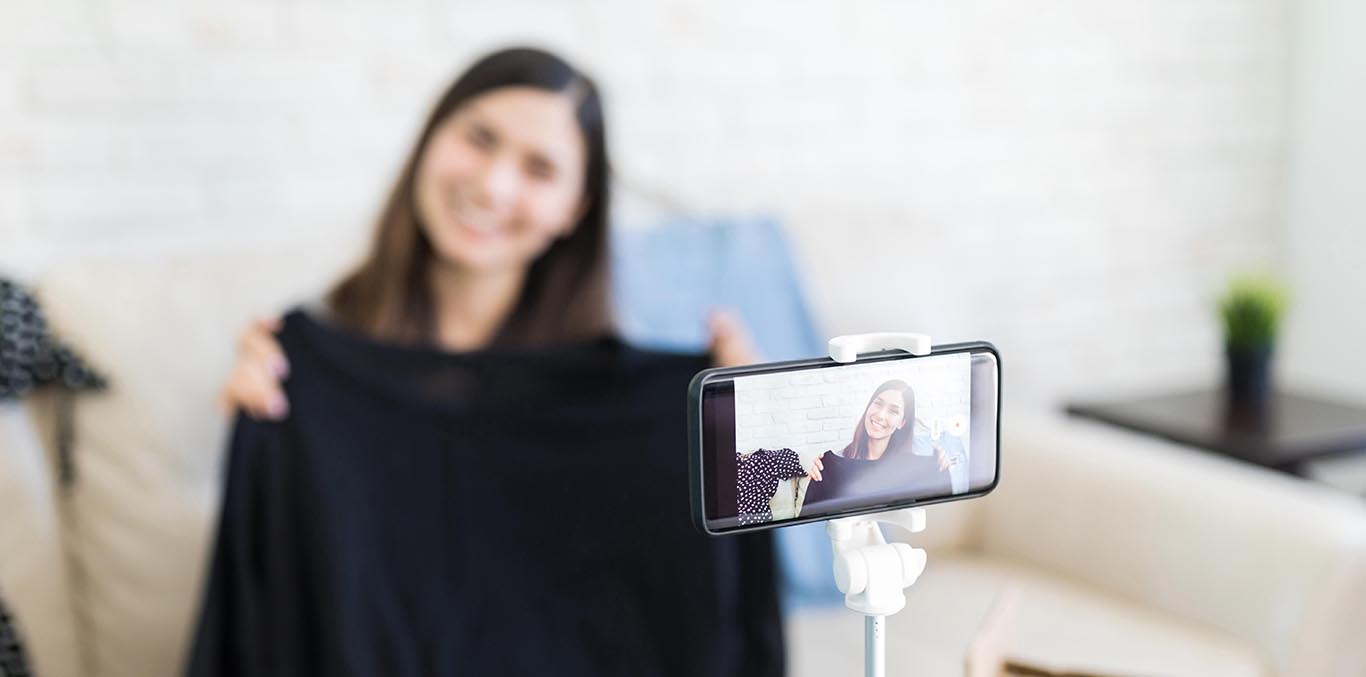
What about weather and social media?
Our experience indicates that most companies are dramatically underusing their own “basic” historical data. Most demand forecasts are not even leveraging the customer loyalty data, and most purchase orders are made without a formal lead time forecast. Thus, while we do not disregard taking clues from external data sources like social media or weather data, we firmly believe that companies should start by making the most of the data they already have.
Scaling up to the challenge
Fashion at scale involves hundreds of stores, and tens of thousands of variants. Therefore, the forecasting engine needs to be able to scale up to millions of SKU positions. Our forecasting engine was natively designed for cloud computing. Unlike traditional solutions, the cloud is not an afterthought for Lokad: Lokad can process terabytes of data daily.
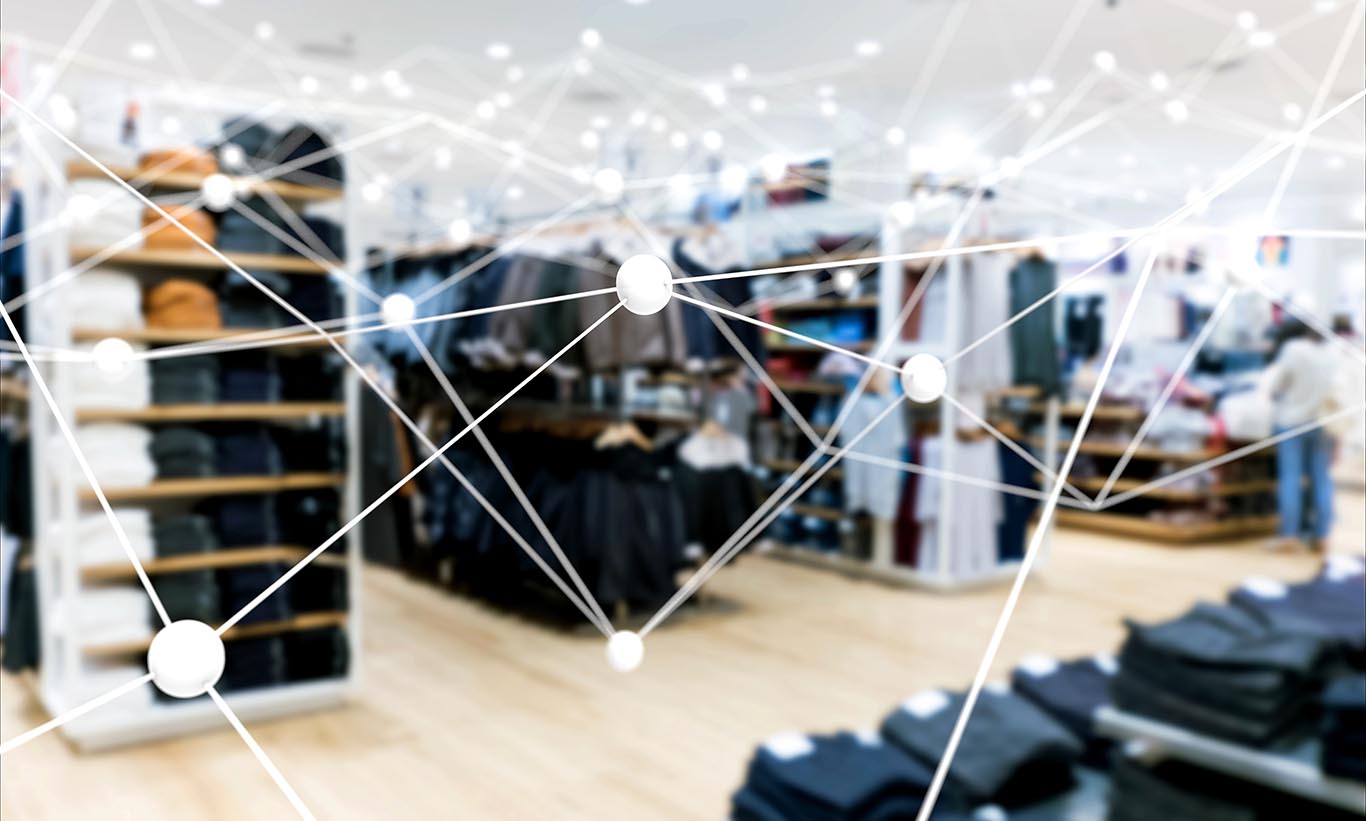
Additionally, unlike traditional approaches, our forecasting capability does not rely on manually linking older and newer products by telling the system which older product should be deemed as the most relevant for forecasting the new product.
Instead, our forecasting engine relies exclusively on advanced machine learning algorithms to automatically detect the similarities that may exist between products, and to identify, by itself, which specific products are relevant for forecasting a new product in the collection.
This automatic detection of similarities relies on the numerous product attributes that are typically present in fashion: product type, product family, size, color, fabric, style, price point, brand, etc. While one might be worried about the amount of data required, our experience at Lokad indicates that catalog data, as it exists for operating the facets of an e-commerce front-end for example, is usually enough for achieving good results.
Traditional forecasting solutions that are based on manual pairing between products are too time-consuming to be effective - there are too many pairs to consider - since it is precisely the pairing that happens to be the main ingredient of the forecasts.
Due to the ineffectiveness of this method, companies tend to revert back to their spreadsheets as the manual pairing forecasting solution fails to deliver the necessary value. Lokad tackles the challenge head-on, focusing on the core difficulty of the challenge, instead of passing the burden on to the users.
However, at Lokad, while we can refer to this forecasting process as product pairing, we do not assume that there is a 1-to-1 mapping between products from an old and a new collection. For example, one product may be split into multiple variants, which may generate cannibalizations. Then, another product might be truly “new”, with no close-matching past products. In such case, the forecasting engine falls back on broader considerations, such as the product category, family, brand or price point.
Economic drivers and whiteboxing
Decisions must be optimized against their expected returns expressed in dollars or euros, not percentages.
All the factors that shape the cost and reward of a decision are collectively referred to as economic drivers: they include the gross margin, inventory carrying cost, transportation cost, shelf space opportunity cost, customer goodwill, etc. Lokad modelizes explicitly all these economic drivers, taking into account the specifics of the fashion market.
For example, whenever a product is sold with a discount, it creates an expectation from the client to benefit from a similar discount in the future.
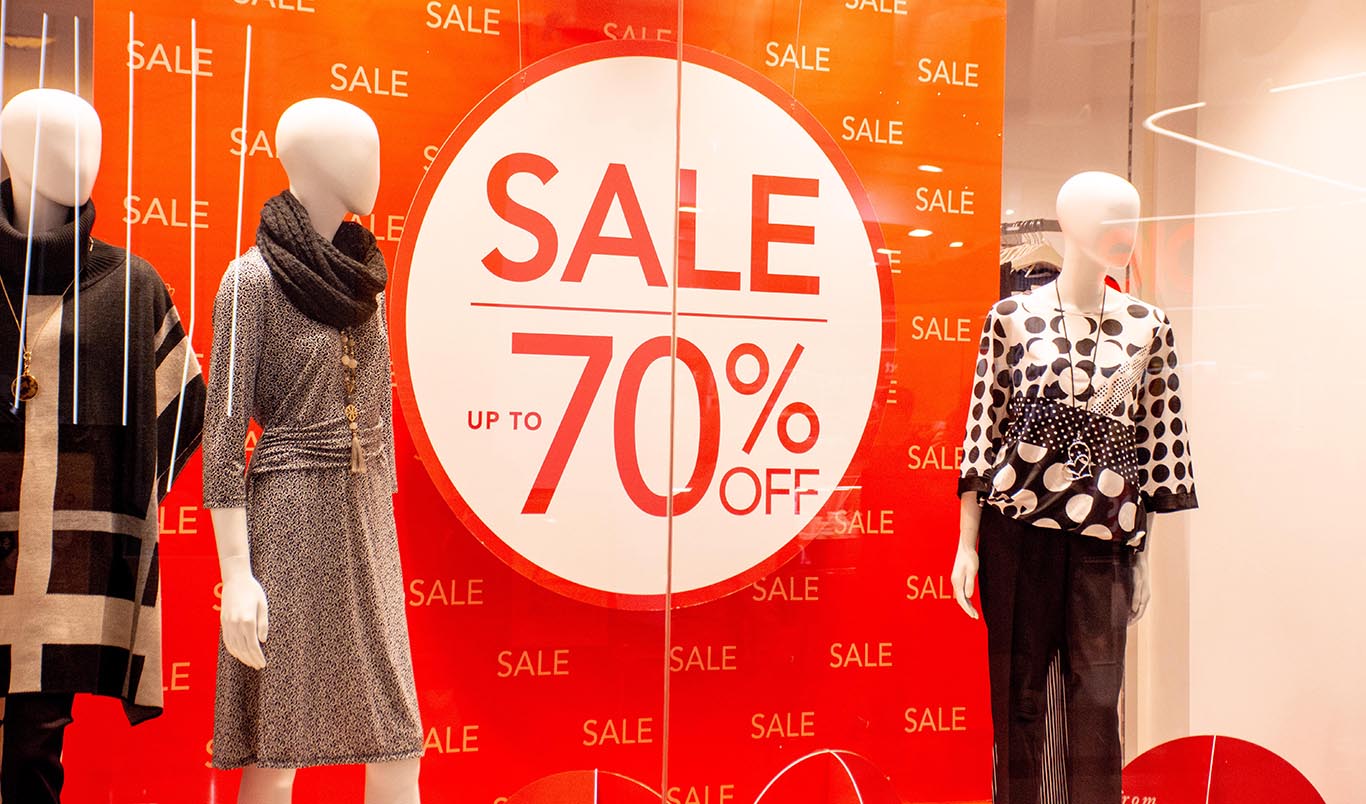
The first purpose of those economic drivers is to support the numerical optimization process that generates the decisions (e.g. purchase order quantities or product discounts). There is no optimization without measurement: the economic drivers are literally what makes the optimization process work.
Lokad is not a substitute for deep strategic insights (those come from your team’s expertise), our technology is merely designed to make it possible to deploy at scale those strategic insights - as reflected through the economic drivers - in every single decision taken by the company.
The second purpose of those drivers is the “whiteboxing” process. The purpose of the whiteboxing process is to deliver to your teams a high degree of transparency for every single decision generated by Lokad. Your team needs to understand why this decision is suggested by Lokad. In order to achieve that, every decision generated by Lokad comes with its own set of metrics - measured in euros or dollars - which explain the business intent of the decision. Those metrics are the different reflections of the economic drivers. Our experience indicates that this approach is superior to trying to shed light on the algorithmic fine-print of the calculation, that is not only incredibly tedious, but also largely nonsensical except to machine learning experts or numerical optimization experts.
From a management perspective, economic drivers are a powerful mechanism to steer the company in the right direction. These drivers are not seen as “immutable” but on the contrary are actually always susceptible to change, in order to reflect the evolution of the market. Lokad’s technology is designed to provide a high degree of agility: with limited efforts, it is possible to elaborate complex what-if scenarios portraying various alternative strategies.