FAQ: Inventory Optimization
Lokad addresses inventory challenges by using predictive analytics to embrace supply chain uncertainty and optimize decisions with financial insights. It considers unique client constraints and enhances decision-making transparency through detailed reports and intuitive dashboards.
Intended audience: Supply chain practitioners, experts, and executives.
Last modified: January 30th, 2024
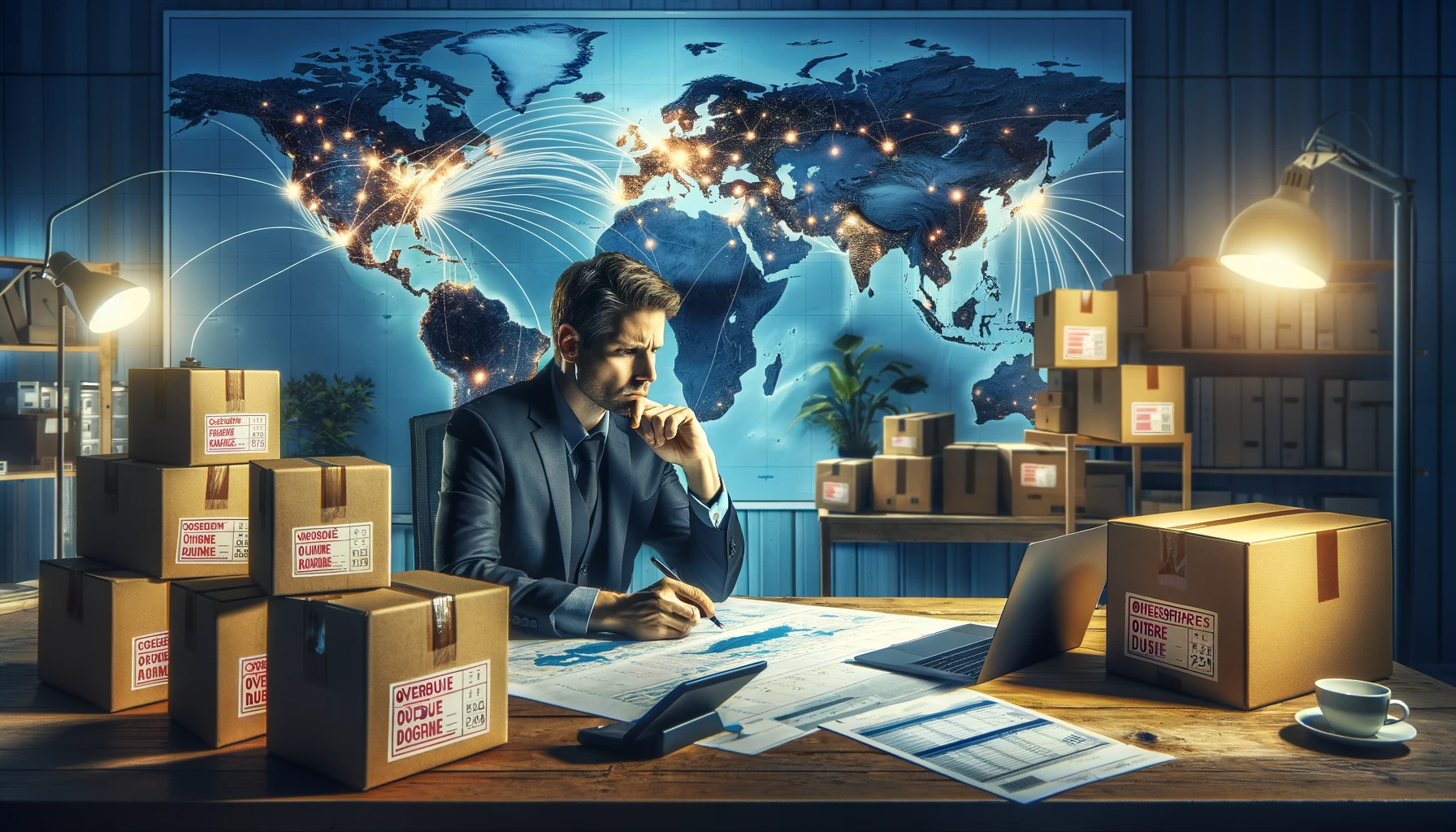
Overstocks and stockouts are the two most obvious symptoms of inadequate inventory-related decisions, and these problems can be traced back to policies that ignore uncertainty. This uncertainty takes many forms, including varying demand, fluctuating lead time(s), and sporadic returns. Lokad delivers superior inventory performance precisely because its programmatic predictive capabilities embrace supply chain uncertainty rather than ignoring it. For example, Lokad has probabilistic modeling capabilities to reflect all the economic drivers connected to a client’s mundane, repetitive supply chain decisions (e.g., purchase orders, production orders, stock allocation, etc.). This allows us to provide clients a high-resolution financial perspective on the dollar (or euro) value of every decision. Lokad also has stochastic optimization capabilities to ultimately recommend the decisions that maximize the dollar (or euro) return for every decision, all while factoring the client’s unique constraints. Such constraints include cross-product concerns like cannibalization and substitution.
At every step of the process, Lokad’s extensive, customized reporting capabilities provide the client complete transparency. Intuitive dashboards are crafted to clearly express the KPIs clients want and need, and to break down Lokad’s calculations in a no-nonsense way.
Executive summary
As far as inventory optimization is concerned, Lokad diverged from what could be considered the ‘mainstream’ approach more than a decade ago. This divergence has only grown as our technology has improved. This technology allows Lokad to effect significant improvements to client supply chains, however the greater challenge is often explaining the improvements – a task made all the more difficult given how different Lokad’s approach is to what supply chain practitioners expect.
The mainstream fairy tale about inventory optimization goes like this: the (magic quadrant) software vendor delivers 20% more accurate forecasts, and those forecasts will be turned into 20% less stockouts and 20% less stock. This fairy tale typically features several topical buzzwords: artificial intelligence, demand sensing, machine learning, blockchain, digital twins, in-memory systems, etc. However, this mainstream view is a dead-end1. Unlike most of our competitors, Lokad does in fact deliver state-of-the-art forecasts2, and we know this is - by itself - nowhere near enough to deliver a satisfactory return on investment.
Lokad is perhaps singular in that we make little mystery of how we achieve superior inventory performance. We have a public lecture series3 (totaling 50+ hours and counting) that provides the fine print of our technologies and methodologies. This series serves as both a resource and a warning for clients: once you see how a vendor’s “tricks” work, you cannot un-see them.
Probabilistic forecasting
Probabilistic forecasts must be used. These forecasts provide a direct quantitative assessment of the uncertainty one contends with in supply chain – e.g., uncertainty surrounding customer demand and supplier lead time(s). Instead of pretending traditional time-series forecasts (the “classic approach”) will spontaneously become accurate, probabilistic forecasts directly address these issues. Probabilistic forecasts are not necessarily more “accurate” - at least not by design – but this is somewhat irrelevant given they are used to tell a completely different story about client’s future – details of which are covered in Financial Perspective. Furthermore, each source of uncertainty deserves its own forecast, not just demand. Lokad produces probabilistic forecasts for lead times, returns, scrap rates, etc.
In contrast, the mainstream time-series forecasts approach ignores the uncertainty described above. As a result, when point time-series forecasts are used, the supply chain is fragile even against risks that are entirely mundane, such as the hit-or-miss nature of product launches. It is pointless to expect that product launches will ever be de-risked, because if a company is able to more reliably assess the performance of its product launches, it would doubtless leverage this newfound capability to launch even more products, thus re-introducing uncertainty to the supply chain in question.
Automated decision-making
Automated (and superior) supply chain decision-making is the only endgame worth pursuing, as these are what tangibly impact the supply chain. If the inventory optimization software returns anything but the finalized decisions, then the software is not doing its job (or you might just have the wrong software).
Moreover, these automated decisions must factor in all the client’s constraints, such as MOQs (minimal order quantities). If one’s software/system keeps generating defective decisions that must be manually overridden, then the system is defective and must be replaced. Real supply chain experts are too scarce and too precious to be used for anything but the continuous improvement of the client’s unique numerical recipe4. Lokad uses this numerical recipe to robotize the supply chain decision-making process in supply chain, thus liberating in-house experts to focus on higher-order issues and strategy.
In contrast, the mainstream view emphasizes all sorts of numerical artifacts: ABC classes (or its cousin, ABC XYZ), inventory turns, safety stocks, economic order quantities, etc. Unlike supply chain decisions, the relationship between a numerical artifact and the performance of the supply chain is fuzzy at best. Moreover, most of these numbers are only introduced in order to support a semi-manual decision-making process, thus ensuring the ongoing waste of client resources (e.g., money, time, and effort).
As a result, nothing ever gets truly fixed as supply chain practitioners are continuously distracted by the persistent firefighting process, and in the background the underlying system continues generating suboptimal decisions.
Financial perspective
The supply chain decisions must be financially optimized, i.e. assessed in terms of euros (or dollars) of impact. This assessment must reflect all the relevant economic drivers. Lokad has access to the relevant, tangible economic drivers (found directly within the client’s ledgers): gross-margin, carrying cost, transportation cost, etc. However, Lokad also quantifies the intangible economic drivers - the kind that must be factored to produce supply chain decisions of value: customer loyalty, the goodwill of suppliers, the perceived value of the brand, etc. Given their abstract nature, these drivers have to be intelligently approximated, though it is far better to be approximately correct than exactly wrong. This monetary value is ultimately used to unify all the different forces that impact every inventory decision.5
In contrast, the mainstream view emphasizes percentages, such as service levels, while wholly ignoring all the intangible economic drivers. This leads to the short-sightedness of recommended decisions that the general public now associate with “finance”. Such decisions are optimized against made-up numerical criteria that simply do not reflect the long-term interests of the business. The fix is not to reject the idea of optimization, rather to revise what is being optimized in the first place.
Frequently Asked Questions (FAQ)
1. Principles
1.1 Do you help streamline inventory management? What are your inventory management capabilities?
Lokad optimizes (and streamlines) inventory rather than managing it. Inventory optimization involves, amongst other things, making the best possible decisions, such as how much stock to buy, when to buy it, and where to (re)allocate it. Inventory management, on the other hand, focuses on managing the electronic records that reflect the physical state of the inventory, and keeping these records aligned with the reality of the inventory. Inventory optimization and inventory management are the two main aspects of overall inventory control, and Lokad is entirely dedicated to the former.
In terms of software design, the requirements of an inventory management system are quite different to an inventory optimization one. In fact, they are often in complete opposition. For example, inventory management requires real-time responses, otherwise mundane operations such as stock picking get delayed until the system finally acknowledges the operation.
However, inventory optimization hinges upon making good decisions, not necessarily real-time ones. A delay of 5 minutes to compute an optimized purchase order - that reflects a wide array of factors and constraints - is inconsequential if the purchase order ends up saving the client thousands of dollars (or euros). From the client’s perspective, this is preferable to a scenario in which mediocre purchase orders -can be produced in under 10 milliseconds (i.e., in real-time)—a decision that could result in losing thousands of dollars (or euros) in dead stock. If the client does not have an inventory management system in place, Lokad strongly recommends installing one before attempting to optimize the inventory.
Note: Most enterprise software designed for inventory management also claim to provide inventory optimization functionality. This is a specious claim. These distinct inventory functions require distinct software interventions.
For a direct comparison of inventory optimization and inventory management, as well as their necessary software, see Inventory Control.
1.2 How do you predict future inventory needs?
Lokad uses extensive predictive modeling technology to forecast all the uncertain factors that impact future inventory needs. These factors include, amongst other things, future demand and future lead times that directly impact inventory needs. However, depending on the specific vertical, there are frequently other sources of uncertainties. For example, there might be customer returns (ecommerce), production yield, scrap rates, etc. In order to establish inventory needs, we typically combine a short series of forecasts that reflect multiple sources of uncertainty. This approach goes far beyond the capabilities of traditional time-series forecasting.
Furthermore, Lokad adopts a probabilistic forecasting perspective. This means that Lokad considers all the possible futures, and quantitatively assesses their respective probabilities. Furthermore, our platform includes the necessary instrumentation to compose or combine these probabilistic forecasts, such as an algebra of random variables (see below). For example, by combining a probabilistic demand forecast and a probabilistic lead time forecast, we get a probabilistic lead demand forecast, which represents the demand integrated over the lead time. The lead demand is typically a starting baseline to assess future inventory needs.
See Probabilistic Forecasting for more information on this perspective. To learn more about our algebra of random variables instrumentation, review Ranvars and Zedfuncs in our public Envision documentation.
1.3 Do you calculate past and future inventory levels based on historic sales data and forecast data?
Yes, Lokad’s platform is capable of calculating both past and future inventory levels leveraging the client’s historical transactional data, including both the outgoing flow (e.g., sales) and the incoming flow (e.g., purchases). We have extensive probabilistic forecasting capabilities, not just for future demand, but also future lead times, future returns, and all other pertinent sources of uncertainty.
Concerning past inventory levels, as Lokad’s solution—delivered through our platform—is both scalable and cost-effective, we usually recommend recording the data over time, typically through snapshots produced within the Lokad platform if this data is not historicized already in the business systems, instead of recalculating this data. Indeed, even a carefully crafted flow model may still incorrectly reflect the past inventory levels. Historical snapshots of the inventory levels do not suffer from this class of problems.
Concerning future inventory levels, there are two important elements to consider. First, the uncertainty of the future is irreducible. Second, future inventory levels depend on decisions that have not yet been made. As the uncertainty of the future is irreducible, it is unwise—and quite unprofitable—to assume that a single projection of the future state of inventory can be deemed “correct enough”. Instead, we should consider all the possible futures, and their respective probabilities. This is much richer view of the future, and allows us to produce risk-adjusted supply chain decisions that are resilient to deviations from the (classic) forecast. Lokad accomplishes this by leveraging probabilistic forecasting.
As future inventory depends on supply chain decisions that have not been made (e.g., future purchase orders), we need a sensible system for implementing decisions in order to assess the possible future state(s) of the inventory. This requires decisions to be automated, so as to permit effective simulation. If the decision-making process is semi-manual (i.e., involving subjective human override), then it becomes impractical to “simulate”. Thus, an automated system of decision-making must be used for simulation purposes.
1.4 Do you calculate Safety Stock, Min/Max levels, Reorder Level, and EOQ (economic order quantity) while factoring in the purchasing policy?
Yes, Lokad’s platform makes it straightforward to implement a mainstream/classic supply chain approach, including safety stocks, min/max levels, reorder points, and EOQs. Moreover, it is also straightforward to have all these elements refreshed in a fully automated manner. However, this supply chain approach is obsolete as it yields poor results in practice. Furthermore, it involves extensive and ongoing manual corrections in order to fix suboptimal outputs that the “system” keeps generating.
In contrast, Lokad recommends a vastly superior approach that consists of assessing the unit-by-unit economic returns for the purchase of each extra unit of stock. This calculation relies on both the probabilistic forecasts that the Lokad platform generates, and on an explicit economic modeling of the consequence of the purchase decisions. This approach embraces the economic drivers that underly the purchase decisions.
As a result, mitigating the purchase overheads is a given: orders are economically optimized, removing the need to introduce obsolete formulas like the Wilson formula (traditionally used for EOQ calculations). Moreover, triggering the purchase order becomes a question of balancing the cost of stockout vs. the cost of overstock, removing the need for safety stocks, min/max levels, and reorder points.
What is more, Lokad’s approach lends itself nicely to cross-SKU (stock keeping unit) concerns. These concerns include cannibalization, substitution, supplier-level MOQs (minimal order quantities), warehouse or store capacities, and multiple echelons. The mainstream/classic supply chain approach is rigidly attached to a strict mono-SKU perspective. As a result, those concerns are dismissed by design. No matter how sophisticated a safety stock calculation might be (to take one example), it cannot address any of those cross-SKU concerns, as the formula (or rather the class of formulas) implies processing SKUs in isolation
1.5 How do you differentiate your approach for high-value items?
Lokad’s quantitative supply chain approach strongly recommends optimizing every supply chain decision with respect to its (individual and interdependent) economic drivers, thus high and low value items are optimized in the same way—though, naturally, the fine print of the calculations vary.
The relevant economic drivers naturally include - amongst other things - the costs and gross margin of the inventory items being optimized. High-value items are naturally associated with high costs and high margins—at least in absolute terms. The optimization carried out by Lokad maximizes the dollar-on-dollar returns for the inventory investments: in simple terms, for every 1 USD that can be invested, Lokad picks the one item that comes with the highest rate of return (think ROI).*
Beyond the pure economic assessment, there are other constraints that impact the generation of inventory decisions, such as MOQs (minimal order quantities). However, Lokad’s platform includes all the necessary numerical capabilities to factor both classes of concern into its optimization. All economic drivers - and consequential financial factors - are proposed by Lokad and ultimately validated by the client.
This unified, financially-driven perspective to optimization means practitioners no longer have to navigate a large array of edge cases governed by different policies and concerns. Each item—high-value, low-value, erratic, etc.— is optimized in terms of the financial impact each additional unit will have for the client.
*At scale, Lokad prepares a ranked list of purchasing decisions, not a just single recommendation. The list is ranked in descending order, starting with the item that carries the greatest financial return on investment. See our public demo account tutorial for a detailed explanation of how this is done.
1.6 Do you factor in PO (Purchase Order) handling costs (e.g., ordering, invoicing)?
Yes, Lokad recommends a financial perspective where every single supply chain decision (including purchase orders) is optimized with respect to all its relevant economic drivers. In particular, all the overheads associated with carrying out the decisions, such as the handling costs, are integrated into this list of economic drivers. Lokad’s Supply Chain Scientists Lokad are responsible for making sure that the economic model, as implemented in the numerical recipe, reflects all the client’s opportunities and overheads.
Lokad’s platform is remarkably expressive and programmatic, allowing us to engineer support for practically any kind of cost and/or discount that may arise in the ordering exercise - e.g., price breaks. Moreover, Lokad’s stochastic optimization allows us to optimize decisions despite the nonlinearities that those costs (or opportunities) typically represent. For example, handling costs may vary little depending on the ordered quantity, which, all things considered equal, should nudge the optimized ordering process toward (slightly) bigger and (slightly) less frequent orders as the handling costs grow. Price breaks also tend to nudge the optimization towards bigger and less frequent orders.
1.7 Can the procurement team simulate the effect of replenishment strategies on capital requirements? Can they project inventory levels?
Yes, Lokad’s platform has been engineered to support projecting, in a very broad sense, future supply chain conditions under varying policies. The future conditions include future inventory levels, but also all the other critical future conditions of the supply chain such as the saturation of the capacities (such as transport, manhandling, storage, etc.). The varying policies include alternative replenishment strategies, but all the other sorts of decisions that govern the execution of the supply chain (stock allocations, production orders, returns, price changes, etc.).
Moreover, Lokad believes it is critical to install a process that leans heavily toward completely automated decision-making (for the mundane repetitive supply chain decisions). It is unreasonable to expect a “simulation” could produce sensible figures if, in practice, the actual execution of the supply chain depends on regular subjective (and manual) overrides from supply chain practitioners. By design, the simulation is entirely ignorant of these overrides.
General probabilistic forecast—not just demand forecasts—are another critical ingredient to accomplish such a simulation. Future lead times, future returns, and many other factors that contribute to future uncertainty must be forecasted. If not, the simulation will operate on the premise of erroneous and unreliable numbers (i.e., data that are blind to other crucial sources of uncertainty). The integration of generalized probabilistic forecasting allows us to construct meaningful simulations that provide the necessary granularity and insight into the client’s supply chain.
See Probabilistic Forecasting for more information on Lokad’s forecasting perspective.
1.8 Can you describe the economic indicators you use to support purchasing recommendations?
Executive summary: Lokad’s programmatic platform enables the incorporation of a wide range of economic drivers into purchasing recommendations, including both direct (first order) like gross-margin and shipping costs, and indirect (second order) such as stock-out penalties and customer goodwill. This programmability surpasses traditional enterprise software, which often limits users to a predefined set of first order economic indicators and lacks the flexibility to address the complexities of real-world supply chains.
We recommend taking into account all relevant economic drivers, which tend to vary (somewhat) from client to client. In general, the pertinent drivers fall into two broad categories referred as first and second order drivers. First order drivers includes all the benefits and costs that can be read more or less directly from the client’s ledgers: gross-margin, shipping cost, carrying cost, ordering cost, etc. Second order drivers are more nuanced, less direct, and entirely absent from traditional enterprise software. These include the cost of not having something on the shelves when a client wants it (stock-out penalty), the goodwill or loyalty of the customer base, the relative importance of customer segments or geographies, etc.
Lokad’s platform has been engineered to make it possible to factor virtually any economic driver; if such a driver can be expressed in a spreadsheet, then it can be expressed through Lokad’s technology. In practice, Lokad’s Supply Chain Scientists are responsible for proposing an exhaustive list of the client’s relevant economic drivers. This list is subsequently validated and/or amended by the client.
This extensive programmability far exceeds the functionality one finds in enterprise software, where the client is presented with a hard-coded shortlist of first order economic indicators to support purchasing recommendations. Such tools are, in practice, unable to cope with the nuances and variety of situations that arise in real-world supply chains. As a result, when the enterprise software relies on such methods, supply chain practitioners invariably revert to previous methods (typically spreadsheets) in an attempt to factor a driver that was absent in the ERP’s shortlist.
On the topic of economic indicators, there is no work-around for full programmatic capabilities. Though spreadsheets are indeed programmatic, they lack the correctness-by-design and scalability of Lokad’s platform.
See The Quantitative Supply Chain in a nutshell for more information on Lokad’s supply chain philosophy, as well as this summary for more information on first versus second order drivers.
1.9 Do you give inventory reports with root causes?
Yes, Lokad’s platform has extensive capabilities for identifying the root causes of the present state of the client’s inventory.
It is worth highlighting that identifying ‘root causes’ is a nontrivial task. It invariably requires extensive work from Lokad’s Supply Chain Scientists to properly identify something that genuinely qualifies as a root cause. For example, overstocks can be traced back to excessive MOQs (minimum order quantities). This can, in turn, be explained by badly negotiated terms from the procurement team. However, the suboptimal terms might be the result of inflated expectations of demand based on an expected evolution of the client’s offering, however the evolution has been postponed due to delays from the marketing team. As such, it can be very difficult to separate correlation and causation, particularly in supply chain.
Fortunately, Lokad’s platform is programmatic. This is a critical requirement when facing open-ended challenges such as identifying root causes. Bugs in the system producing faulty data, practitioners ignoring or manually tweaking recommendations, miscategorized products being allocated the wrong seasonal profile, and basic human error (to name only a few) can all qualify as root causes. Without programmatic capabilities, there is no hope of being able to pursue such inquiries.
Many enterprise software boast RCA (root cause analysis) capabilities or reports but, in fact, they typically identify obvious symptoms and not the actual root causes. For example, if the software identifies an excessive purchase order as the root cause for an overstock, this is not especially helpful if the software recommended the purchase order in the first place. This is particularly harmful if the implicit expectation is that practitioners will manually override the faulty purchase orders generated by the software.
In contrast, any root cause traced back to the numerical recipe Lokad generated for its client would immediately trigger intervention from the supply chain scientist managing the account—typically a corrective rewrite. This rewrite functionality is only possibly because Lokad’s platform is programmatic.
1.10 What analytics and reporting capabilities do you have to track inventory performance and trends?
Lokad’s platform has extensive programmatic analytics and reporting capabilities. This means that any analysis–and inventory performance analysis in particular–that can be done in a spreadsheet or business intelligence tool can also be done in Lokad’s platform.
Furthermore, Lokad’s platform is both scalable and cost-efficient while scaling. This means it is possible for us to historicize everything, including the data that are not always historicized in the business systems (e.g., historical stock levels and historical prices). The platform also adopts a predictive stance with regards to the inventory performance. Indeed, deciding whether there is too much or too little inventory depends on the expectations of future demand. These predictive capabilities are also programmatic, meaning they can be adapted as needed.
Beyond these raw platform capabilities, the recommended approach of Lokad as far as inventory performance is concerned is twofold. First, the performance must be traced back to the original supply chain decisions that generated the stock (or lack of stock). Rather than a root cause, stock levels are a symptom of the action taken by the client (and its supporting software vendor), regardless of whether the action is right or wrong. Second, the performance must be assessed in terms of financial impact (e.g., in euros or dollars), instead of arbitrarily-determined percentages connected to KPIs (e.g., service level).
Financially-based assessments are critical to effectively balance the numerous factors that contribute to every single supply chain decision. Lokad’s platform streamlines these practices, making it easier for analysts client-side to understand their inventory performance—leveraging any number of customizable dashboards available through their Lokad account.
1.11 Do you provide TOP P/N (Part Numbers) lists per category to highlight where the biggest potential for improvement is?
Executive summary: Yes, Lokad’s platform can generate Top P/N lists per category, region, and time-period, and assess improvement potential in monetary terms, emphasizing actionable calls-to-action over simple SKU prioritization. However, Lokad advises against focusing solely on SKU-level performance improvement, preferring the systematic enhancement of the numerical recipes governing supply chain decisions, with SKU-level reporting as a supporting tool.
With Lokad’s platform, it is very simple to generate Top P/N lists of part numbers (or SKUs—stock keeping units) per category, region, time-period, etc. As Lokad’s solution is programmatic, any list that can be crafted in a spreadsheet or a business intelligence tool can also be done in Lokad’s platform. Moreover, by combining our predictive capabilities with our financial perspective, the improvement potential can be assessed in monetary terms (e.g., euros or dollars) instead of arbitrarily-determined percentages connected to KPIs (e.g., service level).
Lokad’s preferred approach consists of computing prioritized call-to-actions, with priorities expressed in Dollars or Euros gained if the proposed item is undertaken. These calls-to-action are diverse and may include expediting or postponing a purchase order, identifying an alternative supplier, increasing the assortment with more variants, phasing out products from the assortment, etc. Unlike a simple prioritization of P/Ns, a prioritization of calls-to-action is, by design, fully actionable. The call-to-action is not necessary expressed at the P/N level. Lokad is capable of expressing very diverse calls-to-action at any granularity, not just P/N.
However, underneath this question, there is a perspective that Lokad does not recommend for supply chain. The intent behind seeking performance improvement at the SKU level is to prioritize the attention of supply chain practitioners in a context where SKUs are expected to be manually reviewed. This approach is obsolete and does not make a proper use of the time of the supply chain practitioners. Inventory problems encountered with a given SKU are almost invariably not specific of this SKU. Instead, there is something wrong with the numerical recipe in general, and unless this more general problem is addressed, next time, the problem will manifest itself through another SKU.
Thus, the time of the experts should be invested in the systematic improvements of the numerical recipes that govern the supply chain decisions. While SKU-level reporting capabilities are important, they mostly matter as supporting instruments for the continuous improvement of the numerical recipes, not as attention-prioritization tools.
2. Stock levels & service levels
2.1 Do you provide inventory-on-hand reports?
Yes, Lokad’s platform makes it straightforward to get a report that covers stock-on-hand levels. Lokad is able to process relational data from the client’s business system to generate such reports. Moreover, Lokad’s platform can also cope with the subtleties associated with stock-on-hand levels, such as reserved stock or backordered stock. All that data can be historicized by Lokad, even if this data is not historicized within the original business systems. Finally, this information can be either presented in eaches or in financial units in line with any valuation rules the client prefers. The inventory-on-hand reports are based on the last incremental synchronization with the business systems.
Such functionality, though, is not Lokad’s primary application. Though Lokad can check real-time stock-on-hand levels for any SKU, our technology is intended as an analytical layer on top of transactional business systems. Those systems remain in charge of ‘managing’ the stock for all the mundane transaction operations, whereas Lokad is designed to optimize inventory decision-making intelligence.
2.2 How do you compute and optimize the inventory service level?
Executive summary: Lokad delivers optimized inventory service levels by integrating probabilistic forecasting with stochastic optimization. This approach enables supply chain decisions to be tailored to specific quantitative criteria, such as balancing high service levels with minimal stock and maximized ROI.
Lokad optimizes inventory service level by combining probabilistic forecasting and stochastic optimization capabilities. This allows us to optimize supply chain decisions to reflect any quantitative criteria that could be expressed in Excel, only better. In particular, optimizing replenishment decisions in line with certain service levels—while minimizing the amount of stock and maximizing ROI— is very straightforward.
Probabilistic forecasts are extremely powerful for making sure supply chain decisions truly reflect the intended service level, even when the service levels are very high (e.g., 98% and higher). Classic time-series forecasts (i.e., non-probabilistic) and their classic inventory methods (e.g., safety stocks) systematically fail under these conditions, as the underlying model assumptions (i.e., normal distributions for demand and lead times) are systematically violated by real-world supply chain conditions. Lokad can, and occasionally does, optimize service levels. In order to do that, we introduce the relevant economic drivers: carrying costs, capital costs, stock-out penalties, gross-margin, etc. Then, we compute the service levels that maximize dollars-of-return per dollar spent by the client.
Once this is done, our clients usually realize that service levels can, in fact, be entirely side-stepped. Lokad has the ability to leverage the aforementioned economic drivers to directly optimize supply chain decisions themselves (rather than somewhat arbitrary supply chain KPIs). Thus, inventory ends up having service levels that reflect the most profitable strategy for the company. This is true despite the fact Lokad directly optimizes dollars-of-return per dollar spent rather than percentages connected to arbitrary KPIs.
“Arbitrary KPI” is not hyperbole. First, service level is typically predicated on meeting customer requests in isolation, rather than meeting customer requests in the most profitable way possible. The latter is a much more nuanced perspective that accounts for the associated costs of trying to meet high service levels for SKUs that might not, in and of themselves, be especially profitable. Ignoring this perspective ensures the continuation of unnecessary inventory costs (and inventory write-offs). This is because arbitrarily high service levels generate, by design, an ongoing flow of dead inventory.
Second, service level—an arbitrary, bureaucratic percentage—does not equal “quality of service” for customers. Service levels ignore, by design, all the cannibalizations and substitutions that exist in one’s offering. Conversely, service levels also ignore, by design, all the dependencies that exist within the offering, where getting product A only makes sense if product B is jointly available.
Third, even from a single SKU perspective, service levels do not address bulk orders—situations where a customer expects a certain quantity to be available for purchase. In this event, both empty shelves and insufficiently stocked ones are threats to quality of service.
In short, service level is an obsolete supply chain instrument, not to be confused with ‘quality of service’, which remains as important as ever. Lokad acknowledges that timely change management may require a transition period featuring such KPIs, however, in the long-term we strongly recommend the superior economic perspective that optimizes the dollars-of-return per dollar spent.
See The Quantitative Supply Chain in a Nutshell for more on Lokad’s financial perspective.
2.3 How do you optimize stock levels and reduce carrying costs?
Executive summary: Lokad follows a two-stage process. First, we establish a probabilistic predictive model. The predictive model is a generalized version of the old (now obsolete) time-series forecasting models, as it covers all sources of uncertainty, not just demand. Second, we apply a stochastic optimization. The stochastic optimization is the process that outputs the supply chain decisions of interests, e.g., replenishment quantities. The optimization is said to be ‘stochastic’ as the optimization criteria is noisy/randomized, something that reflects the uncertain future supply chain conditions.
Lokad’s preferred optimization criteria reflects the client’s economic drivers. Thus, when optimizing a client’s inventory, Lokad explicitly quantifies the various costs (e.g., carrying costs, working capital costs, write-off costs, etc.) as well as the upside (e.g., gross-margin, stock-out penalties, etc.) to maximize profitability. As such, we adjust stock levels to minimize carrying costs, but only to the extent that those gains are not offset by losses arising from degraded quality of service.
Though Lokad’s preferred optimization criteria is rooted in the meticulous quantification of the client’s economic drivers, we can adjust our criteria to reflect any number of alternative factors. Lokad’s platform is programmatic, meaning it can be adapted to satisfy any criterion the client desires, including any that could be expressed in a traditional Excel spreadsheet.
See Economic Drivers in Supply Chain and The Quantitative Supply Chain in a Nutshell for more on Lokad’s financial perspective.
2.4 How do you optimize safety stocks to minimize stockouts while controlling holding costs?
Executive summary: Lokad’s risk-adjusted decisions carefully minimize the actual financial risk of stockouts by raising the stock level(s) to the point where the marginal holding costs are greater than the cost of a stockout event. Given the optimization needs to contend with constant ambient uncertainty—the future is unknowable—stochastic optimization is required. Traditional forecasting and optimization methods (i.e., deterministic models) cannot cope with the noisy/randomized future variables—probabilistic forecasts with stochastic optimization can.
Lokad optimizes all stocks—including safety stocks—by leveraging probabilistic forecasts combined with a stochastic optimization. The probabilistic forecasts include future demand and future lead times, as well as any other relevant source of uncertainty. The stochastic optimization can be adjusted to suit any numerical criteria, including minimizing stockouts under a maximal holding costs constraint.
Probabilistic forecasts are uniquely adept at addressing the unusual conditions that generate stockouts in the first place. If a stockout happens, it is typically because either demand or lead time has surged unexpectedly—possibly both. Classic time-series forecasting models (i.e., non-probabilistic) only identify a single future value (e.g., X), thus dismissing entirely what it deems the less likely—though far from improbable— alternative values (e.g., X+1, X-1, etc.).
As a result, classic time-series forecasts are largely blind when it comes to quantitative assessment of unlikely events, like the one that would cause a stockout. When a company aims to have a stockout frequency of less than 1% of the time (e.g., per quarter), the stockouts that still happen are, by design, part of the top 1% most extreme situations.
The stochastic optimization process is essential for turning the original probabilistic forecasts into supply chain decisions, such as inventory replenishments. Lokad’s preference is a pure economic approach where the cost of stockouts is expressed in dollars (or euros), alongside the other costs, such as the holding (carrying) costs.
In Lokad’s estimation, the safety stock model is an obsolete concept, though it can be included in our platform at the client’s request. We strenuously recommend fully embracing the quantitative supply chain perspective, rather than relying on methods that are not fit for purpose. For example, arguably the biggest defect of safety stock models is that (by design) they cannot prioritize, in the event of two SKUs on the verge of a stockout event, which one is the most important. This perspective treats SKUs in strict isolation, thus defeating the attempt to optimize the supply chain as a whole.
See Why Safety Stock is Unsafe and Retail stock Allocation with Probabilistic Forecasts for more on these points.
2.5 Do you dynamically calculate and adjust safety stock levels at the store/warehouse level?
Executive summary: Yes, Lokad’s platform allows for the entire inventory optimization process to be refreshed whenever the new input data is provided—typically on a daily basis. As a rule of thumb, we keep all the calculations under 60 minutes, including refreshing all forecasts and decisions for every single SKU at every location—stores and warehouses included. This flexible and scalable approach is only possible due to Lokad’s specific engineering decisions.
Lokad’s platform emphasizes a ‘stateless’ design for supply chain optimization. We do not recycle previous calculations, rather we recompute everything every time we receive raw input data. Though this can increase computing resources, it is the only effective method (currently available) for ensuring the integrity of data processing—something that is compromised without Lokad’s stateless design. The alternative is allowing the client’s production environment to become the testing ground for semi-verified data—something Lokad does not recommend.
These refreshes cover all the settings that govern the supply chain optimization Lokad delivers. If desired by the client, the refreshes can include time-series forecasts, safety stocks, and optimized service levels. However, Lokad strongly recommends clients not to depend on these obsolete methods, and to fully embrace the superior optimization power of probabilistic forecasting and stochastic optimization.
Please consult Refresh Everything Every Day for more on this point.
See also Stock levels & service levels 2.4 in this FAQ.
2.6 Do you evaluate the impact of safety stock levels/formulas on maintaining desired service levels?
Yes, through Lokad’s platform a supply chain practitioner can evaluate the impact of a given safety stock on the service level. In other words, one can pick a safety stock level and see the corresponding service level. The platform also lets the supply chain practitioner evaluate other factors, such as the expected carrying costs (or at least holding durations if the economic characteristics of the stocks have not been provided), and/or the risk of dead stock.
However, safety stocks and service levels are largely obsolete concepts. Despite being capable of including them in our platform, Lokad does not recommend either of them. Probabilistic forecasting and stochastic optimization—the bedrock of Lokad’s optimization—represent a superior alternative in every dimension.
Please consult Retail Stock Allocation with Probabilistic Forecasts for more on this point.
See also Stock levels & service levels 2.4 in this FAQ.
2.7 Does Lokad allow for the implementation of varied inventory strategies, each with specific service levels and confidence intervals, differentiated across multiple organizational dimensions (e.g., by SKU, product category, and/or region)?
Executive summary: Yes, Lokad’s platform supports differentiating inventory strategies or policies at the SKU level. Such strategies can reflect differentiated settings—at the SKU level—considering varying service level targets, varying confidence intervals (for safety stocks), etc. These strategies can also be differentiated at any intermediate granularity (not only the SKU level). For example, it is possible to selectively apply settings per region, per product categories, per item attribute (e.g., threshold on item weight), etc.
Lokad’s platform is programmatic, hence we can implement any inventory strategy that could be expressed in a spreadsheet—regardless of how arbitrary or unusual this strategy might be. Lokad’s Supply Chain Scientists carry out these tasks and ensure that the outcome faithfully reflects the original intent of the client company.
Though Lokad’s platform can be used to pursue service levels, we strongly recommend not doing so. Lokad’s platform is best dedicated to optimizing dollars-of-return per dollar spent, not percentages connected to arbitrary KPIs. In fact, there are two broad objections to the very notion of ‘service level’.
First, despite a similar name, the connection between service level and quality of service—as perceived by the consumer—is tenuous. Many supply chain textbooks, and consequently many supply chain software, falsely conflate the two notions. Service levels entirely ignore the range of all possible substitutions, as well as all possible dependencies between products. Service levels are, as such, a poor proxy for an actual understanding of quality of service.
Second, service levels, by design, contribute to the ongoing generation of inventory write-offs. This is on account of its singular focus on the upside (i.e., servicing demand), which ultimately means it is blind to the downside (i.e., being left with units of stock that never get sold/requested/consumed).
2.8 Can you enforce service level and inventory turns by SKU or by P/N (Part Number)?
Yes, Lokad’s platform allows us to tune the inventory optimization process toward a given service level/inventory turn, down to the SKU-level, or at any intermediate granularity level (P/N, brand, location, category, price point, etc.). In this regard, Lokad’s platform can direct inventory decisions to reflect any quantitative target(s) the client desires.
However, no vendor can promise that their settings will be ‘enforced’, at least not on a given SKU. Fundamentally, both service levels and inventory turns depend on the behavior of the customers. If there is a surge of interest for a given product, the resulting demand may vastly exceed stock levels, and the service level target will not be met. Correspondingly, if there is a drop of interest, then inventory turn targets will not be met, either.
Lokad’s probabilistic forecasting technology does ensure that, on average, across many SKUs (i.e., thousands) and over a sizeable period of time (i.e., weeks) the observed service levels and inventory turns reflect the client’s desired settings. This is, practically speaking, the “closest” one can reasonably come to “enforcing” settings that are ultimately determined by the customer(s).
See also Stock levels & service levels 2.7 in this FAQ.
3. Overstocks
3.1 How do you integrate the risk of inventory write-off?
The probabilistic forecasts used by Lokad are a critical ingredient of the inventory write-off assessment. Through probabilistic forecasts, Lokad assesses all the possible futures, including less likely ones, as opposed to having the whole analysis rest on a single future deemed value (i.e., classic point/time series forecasts). In reality, it is the expected (unlikely but not impossible) sharp drop of demand that causes inventory write-off, something that probabilistic forecasts are designed to explicitly quantify ahead of time.
Once the probabilistic forecasts are established, Lokad produces risk-adjusted supply chain decisions. These supply chain decisions are optimized with respect to the relevant economic drivers, of which the possibility of inventory write-off is one. The decision (e.g., an inventory replenishment) is risk-adjusted as it balances the upside of better servicing the customers with the downside of possibly being left with dead stocks later. Moreover, Lokad can reflect the intermediate option that may be available to the client company, such as secondary sales channels that can absorb the extra stock, albeit at severely discounted prices.
See also Stock levels & service levels 2.3 in this FAQ.
3.2 How do you handle and optimize dead and/or sleeping inventory?
Lokad proactively addresses dead inventory by eliminating, or at the very least severely reducing, the supply chain decisions that ultimately generate dead inventory in the first place. This proactive mechanism relies extensively on the probabilistic forecasts that Lokad generate.
Unlike classic time series forecasts that identify a single future value—hence ignoring all alternative values—Lokad quantitively assess all the possible futures via their respective probabilities. Through this assessment, we quantify the risk of generating dead stock for every supply chain decision (e.g., ordering 5 units instead of 4). If a supply chain decision (e.g., order 5 more units) is deemed too risky in terms of dead inventory, then this decision is adjusted to lower the risk. Naturally, the risk of inventory write-off cannot be entirely eliminated, but once adequately assessed, the frequency of inventory write-offs can be drastically reduced.
Concerning sleeping inventory, unless this inventory serves a very specific purpose (as might be the case in industrial settings where spare parts address rare but critical failures), Lokad would typically recommend lowering the price in order to boost demand. This would ultimately liquidate what remains of the inventory. Lokad’s platform is capable of producing a joint inventory and pricing optimization process for this very purpose.
See also Overstocks 3.1 in this FAQ.
3.3 Do you identify nonperforming inventory, e.g., E&O (excess and obsolete) and dead stock?
Executive summary: Yes. Using probabilistic forecasts, Lokad identifies and quantifies the lifecycles of the units in stock for every SKU. For every unit in stock, we estimate the probability that this unit will be requested (or served or consumed) within any given time horizon. For example, when considering a SKU that is overstocked, we can assess what fraction of the stock is at risk of becoming dead stock, and what fraction is at risk of becoming deprecated stock (requiring reductions to inspire sales). This is the same approach we take regarding excess and obsolete stock, and is a major ingredient in the production of our recommended supply chain decisions.
The probabilistic forecasting perspective lets Lokad consider all possible futures values (e.g., of demand), instead of relying on only one (as per a traditional time series forecast). This perspective is critical for addressing all the nuanced variations that exist when trying to mitigate inventory risks. Considering dead stock is something that can only be prevented (rather than handled after the fact), the challenge is to install a process that effectively reduces the likelihood of accruing dead stock in the first place. Such a process requires precisely quantifying the probability that a supply chain decision will generate excess/obsolete/dead/deprecated stock.
Time series forecasting models are, by design, incapable of this class of risk assessment. A chief reason for this is time series forecasts only consider a single future value (e.g., demand). This level of simplicity allows for SKUs to be neatly decomposed into tidy categories (such as A/B/C-classes in an ABC Analysis). However, this simplicity means a detailed risk assessment is not possible, given a ranked list of risk-adjusted decisions for any given SKU requires data for multiple future scenarios (i.e., selling 1/2/3/4/5/etc. units of the same SKU).
In short, adopting a probabilistic approach to forecasting (in this case, demand) lends itself to an effective, proactive strategy that mitigates the generation of nonperforming inventory, rather than trying to handle it after the fact—at which time, there is little choice but to dispose of it.
3.4 Do you have KPIs for tracking active vs sleeping vs new inventory?
Executive summary: Yes. Lokad’s platform is programmatic—meaning we can design whatever kind of KPI we desire—and our Supply Chain Scientists carry out the construction and implementation of the client’s KPIs (in conjunction with the client’s input). Lokad provides bespoke KPIs that genuinely reflect the nuances of the client’s inventory. Lokad can also reproduce whatever KPIs the client may have previously used and wishes to retain, though typically these will be less useful than the bespoke ones Lokad designs for the project.
Not only can Lokad track the exact age of every single unit in stock, but also, thanks to our probabilistic forecasts, we assess the probability that the unit will remain in stock for any given duration (1 week, 1 month, 1 year, etc.). Qualifying the inventory as active vs sleeping is not a mere matter of visualization of the historical data. Rather, it involves a projection of future demand. Thus, ‘KPIs’ are predictive. As a result, they implicitly depend on the suitability of the underlying predictive model to carry out such a task. Here, probabilistic demand forecasts are well suited to assess the risks associated with a harmful drop of demand.
In contrast, some enterprise software vendors treat inventory KPIs as if they were a direct reflection of this historical data. However, the ‘predictive’ aspect cannot be avoided. More often than not, those vendors do not realize that they are implicitly relying on a ‘moving average’ demand forecast, hence giving the illusion that no forecasting ever takes place. In turn, those improper KPIs prove harmful for the company, as they merely distract supply chain practitioners.
See also Overstocks 3.3 in this FAQ.
3.5 How do you integrate, monitor, and optimize monthly inventory depreciations? How do you handle variability in the depreciation process itself?
Executive summary: Lokad addresses inventory depreciation through a comprehensive approach involving tracking inventory composition, modeling depreciation mechanisms, and generating risk-adjusted supply chain decisions. By maintaining detailed records of stock levels and ages, using predictive models to anticipate future stock composition, and employing programmatic capabilities to reflect various depreciation mechanisms, Lokad effectively manages both internal and external depreciation factors. Lokad’s platform leverages stochastic optimization to make supply chain decisions that consider all economic drivers, including depreciation costs, thus proactively mitigating inventory depreciation risks and balancing them against other operational considerations.
Addressing inventory depreciations involves addressing a series of subproblems. The first problem consists of keeping track of the exact composition of the inventory—not just of the totals expressed in stock units. For example, the age of every unit matters. The second problem consists of modelling the depreciation mechanism itself. It can be a purely endogenous phenomenon, where the goods degrade over time, or an exogenous phenomenon, where market conditions change and devalue the goods in the process. The third problem is to generate supply chain decisions that are risk-adjusted with regards to the potential depreciation.
First, through Lokad’s platform, we keep track not only of the stock levels, but the stock age of every unit in stock (both past and future). This process is a predictive model of its own. Even when looking at historical data (obtained from business systems), the age composition of the units in stock is usually not recorded, it can only be inferred. There is a notable exception for serial inventory, where each unit in stock is tracked by its serial number; in this case, there is no need for a predictive model for the past, but we still need one for the future. The predictive model for the composition of the inventory can rely on a FIFO (first-in, first-out) assumption for the consumption of inventory, and a few more nuanced patterns.
For example, in a retail store, customers may sometimes adopt adversarial behavior, such as cherry-picking the best-looking goods (or choosing by expiration dates, if available), turning the inventory consumption in a LIFO (last-in, first-out) to some extent. Lokad can manage FIFO and LIFO, as well as the whole spectrum in between.
Second, through the programmatic capabilities of Lokad’s platform, we can reflect any depreciation mechanism. For example, Lokad can reflect an exponential depreciation where goods lose a small fraction of their value at every period; or Lokad can reflect a staircase depreciation where goods lose a sizeable fraction of their value at certain age thresholds. Furthermore, the depreciation mechanism can involve cross-product dependencies. For example, goods can be expected to lose a sizeable portion of their value when superior rival goods are introduced—as frequently happens in certain verticals (consumer electronics for example).
The variability of the deprecation process, which tends to be pronounced when considering exogeneous depreciations, benefits from predictive (probabilistic) models within Lokad’s platform. We do not need to know exactly when rival product will be introduced; by looking at the historical data, we can model the rate of product replacement, and reflect the probability for any given product to be made obsolete withing any given time horizon (e.g., a week, a month, a year, etc.). These probabilistic models are learned leveraging the historical data made available to Lokad.
Third, risk-adjusted supply chain decisions are computed by Lokad, considering all the relevant economic drivers. The process is a stochastic optimization, as the loss function (i.e., costs and benefits) is noisy/varying. Depreciation costs are included alongside all the other relevant drivers. As the Lokad platform features both programmatic learning and optimization paradigms, we can produce risk-adjusted decisions while considering (and mixing) very diverse sorts of costs.
In conclusion, by producing risk-adjusted supply chain decisions (e.g., purchase order, production order, etc.) that take inventory depreciation into account, Lokad proactively mitigates the amount of inventory that will ultimately incur depreciation. Every decision is gently steered away from the depreciation risk, but not so much that the steering would make another problem worse, such as degrading the quality of service beyond what is gained through the reduced depreciation.
3.6 Do you provide a surplus/excess/overstock inventory report?
Executive summary: Yes. Lokad’s platform features state-of-the-art demand forecasting technology. We leverage this technology to assess how much time it will take to cycle all the committed stock, either on hand or on order. Moreover, our technology supports probabilistic forecasts, which provides a direct quantitative assessment of the risks of overstock. By combining probabilistic forecasts with an economic model of the inventory that reflect all pertinent economic drivers, Lokad delivers a quantitative assessment of the inventory risks expressed in terms of monetary impact terms (e.g., euros or dollars).
Stock is always considered ‘excessive’ when compared to a predictive model of the demand. There is no such thing as ‘excessive stock’ without making a statement about the future demand (although this statement can be implicit). Any software solution that generates an overstock report based on rules such as ‘more than X months of stocks’ is, implicitly, basing their overstock assessment on a moving average demand forecast, which proves underwhelming for most verticals.
Furthermore, without a robust economic model to reflect the inventory cost/reward equation, the client company exposes itself to major inventory mistakes. A financially-driven decision regarding inventory may seem odd at first, though be perfectly sensible. For example, if an item is very cheap, very small, sold with a comfortable gross-margin, absolutely required by the customer (in small quantities), and can only be acquired by hitting very large MOQs (minimal order quantities), it might be reasonable to carry more than one year’s supply of this item in stock. While more than 1 year’s worth of stock might look like a mistake, the return on investment may tell a completely different story.
See No1 at the SKU-level in the M5 forecasting competition for more on how Lokad’s approach to forecasting in practice.
4. Stockouts
4.1 Do you allocate inventory for SKUs in risk of out-of-stock status?
Yes, generally speaking, one of the consequences of Lokad’s inventory optimization is that inventory ends up being allocated to SKUs as their risk of stockout increases. All things considered equal, SKUs on the verge of a stockout event receive priority attention.
That said, we recommend adopting an economic, end-to-end perspective for the optimization when it comes to stockouts. This means considering the total financial impact of a supply chain decision (e.g., allocating stock to avoid a stockout event). Occasionally, avoiding stockouts does not always make economic sense.
For example, for fashion stores it is natural at the end of the season to progressively allow the articles of a dated collection to reach stockout status. This is done intentionally in order to make room for the next, newer collection. Similarly, if a product is superseded by a superior alternative, it makes sense to let the obsolete product reach stockout status, and then phase out the product from the assortment. Thus, broadly speaking, a reasonably designed economic optimization would attempt to avoid stockout events.
However, such an optimization would also provide a much more granular and sophisticated response when it comes to properly sizing the investments (e.g., how many units are allocated to a given SKU) in the first place—in order to mitigate financial risk.
4.2 How do you prioritize POs (Purchase Orders)?
Executive summary: Lokad prioritizes Purchase Orders (POs) by evaluating the economic returns of each stock unit to be ordered, aiming to maximize the return per dollar spent. This granular assessment, integrated with cross-SKU constraints like minimal order quantities, is guided by probabilistic forecasting and stochastic optimization. These technologies assess risks and returns for each unit, considering factors like demand, lead times, and returns, to create financially optimized POs that balance constraints and economic viability.
Lokad’s approach to optimization consists of assessing the economic returns for every single stock unit to be ordered. This is done to mitigate risk by maximizing the dollars-of-return per dollar spent. This very granular assessment is then leveraged by an optimization process that generates the POs that correctly reflect all the cross-SKU constraints (e.g., minimal order quantities at the supplier-level). These constraints are overlaid on top of the per-unit economic returns that guide the overall prioritization. As a result, Lokad’s POs are internally correctly prioritized and each one comes with its own economic assessment (i.e. the dollars of return). This economic assessment is obtained through the aggregation of the economic returns for all the units that are bundled in the PO.
This financially-driven prioritization of POs relies on two key technological ingredients: probabilistic forecasting, and stochastic optimization. Both technological ingredients are part of the Lokad platform.
Probabilistic forecasting, which includes forecasting not only the future demand but all the other sources of uncertainty such as lead times or returns, is critical to assess the risks associated with a purchase order. These risks include the upside (e.g., reducing the risk of a stockout event) and the downside (e.g., increasing the risk of dead stock). Through probabilistic forecasting and leveraging the relevant economic drivers (e.g., gross-margin, carrying costs, etc.), Lokad generates the expected risk-adjusted return on investment for every stock unit about to be ordered, including the diminishing returns as more units get ordered.
The stochastic optimization process allows for the composition of the POs themselves. In the absence of constraints, it is a simple prioritization of the units to be purchased—ranked by their respective dollar-on-dollar rate of returns. However, when cross-SKU constraints are present, a stochastic optimization process is required to successfully and automatically compose POs that enforce all those constraints, while preserving the underlying unit-by-unit financial assessment.
4.3 Do you pass/send orders to suppliers regarding strategic/critical SKUs?
Yes, the purchase orders generated by Lokad’s platform integrates all the relevant concerns, including the strategic/critical importance that certain SKUs have. These concerns can feature explicit information from the client as well as implicit/inferred quantitative assessments discovered through Lokad’s analysis.
For example, a SKU might be “critical” due to being primarily sold or serviced to large, important customers. Alternatively, a SKU might be critical as it is part of a kit or a BOM (bill of materials), making this SKU a bottleneck of a kind. For these situations, it is preferable to leverage the Lokad platform to infer the importance of the SKU through direct data analysis.
More generally, the financial perspective Lokad recommends is well-designed to accommodate varied concerns and blend them with the recommended supply chain decisions. This financial perspective emphasizes the integration of all economic drivers—including less obvious ones like the cost of a stockout event—into the supply chain decision-making process.
4.4 How do you consolidate critical POs (Purchase Orders) into a critical shortlist for key suppliers?
Executive summary: Lokad’s approach is to quantify the economic impact of every supply chain decision. In practice, this involves assessing the financial impact (measured by dollar-on-dollar return) for ordering every single additional unit of stock. By generating a list that ranks items in terms of their dollar-on-dollar return, Lokad automatically generates lists that reflect the best possible financial state for the client with respect to their economic drivers and KPIs (including service level). This removes the necessity for traditional methods, such as min/max, safety stocks, etc., which ultimately lack a robust financial dimension.
Lokad’s probabilistic forecasting approach allows us to generate risk-adjusted POs that consolidate all the units that can be profitability ordered (ranked on a unit-by-unit basis). This makes it straightforward to refine the PO by isolating, within the PO, the units that exceed a given level of economic returns. These high-return units are the essence of the shortlist to be communicated to key suppliers. The economic return reflects the stockout penalty (a less common but critical economic driver that measures the financial impact of a stockout event) in one form or another. This is all done in order to properly assess the importance of every single unit when it comes to the intended quality of service.
Some old (now obsolete) methods (still present in some enterprise software) consist of assessing POs in relation to stock level targets (e.g., min/max, safety stocks, cover targets, buffer targets, etc.). However, these methods all lack mechanisms for quantifying the importance of every single unit in strict isolation from the other units.
In reality, such methods do not reflect the influence of diminishing returns one finds in supply chain. Typically, the first unit ordered is worth more (both for the client company and its customers) than the second unit. As traditional methods do not quantify the inventory returns on a unit-by-unit basis, they are incapable of extracting a genuinely critical subset of units to be passed to key suppliers.
Composing a truly critical shortlist requires, by design, a technology that can quantify the economic returns on a unit-by-unit basis when ordering—technology Lokad possesses.
4.5 How do you communicate a critical shortlist, among pending POs (Purchase Orders), to suppliers?
Lokad prefers to communicate POs—including critical shortlists—via transactional business systems (e.g., an ERP or similar business software). The POs typically take the form of flat file exports. Lokad could leverage email, but our preference is to maintain all transactional communication within the client’s business system (e.g., ERP).
See also Inventory Control for more on the distinction between analytical systems (which Lokad fundamentally is) and transactional systems like ERPs.
See also Stockouts 4.4 in this FAQ.
4.6 Do you provide a stockout report?
Executive summary: Yes, Lokad’s platform is capable of presenting a stockout report that covers not only the present state of the inventory, but also a historical view of previous stockouts. This allows us to identify past patterns and factor them into the numerical recipe that generates the client’s financially-optimized POs, thus reducing the likelihood of future stockout events.
While it is easy to count the number of SKUs (stock keeping units) that have zero stock, it does not say much about the impact for the business of the client company—the net loss of demand must be quantified. For this reason, Lokad recommends approaching the problem through a direct financial assessment of the cost of (a lack of) quality of service. Occasionally, for example, it is reasonable to accept low quality of service for non-strategy products being gradually phased out from the offering. Doing the opposite would guarantee an ongoing generation of dead stock. Lokad’s platform makes it possible to assess the loss, expressed in monetary terms, instead of just counting SKUs with zero stock on hand.
The quantified loss must be ‘net’ of cannibalization, substitution, and delayed consumption from the customers. In most verticals there are several options to satisfy what the customer wants or needs. Thus, as long as one of those options is available, the customer might still be very satisfied with the service. Not considering these cross-SKU factors leads to overstocking SKUs that fundamentally serve the same demand at the expense of other SKUs that serve entirely different segments. Conversely, sometimes a SKU being out-of-stock prevents the consumption of another dependent SKU. Customers expect both SKUs to be available and consume neither if it is not the case. Lokad’s platform can be used to model these cross-SKU concerns and their (financial) impact on the quality of service perceived by the customers.
Depending on the vertical, there might be more important subtleties to be considered. For example, in a DIY (do-it-yourself) store, a customer seeking 4 identical light switches is unlikely to buy any if there are only 3 units available on the shelf. Thus, while light switches are not out-of-stock, from the perspective of some customers, they effectively are.
Another example would be a grocery store selling fruit. Certain fruits—e.g., strawberries—are highly perishable, thus the store usually deliberately attempts to hit the stockout before closing. Yet, if the stockout is hit too early in the day (e.g., a few individuals purchase more than average), the bulk of customers are not properly served.
These examples highlight the nuance behind the nature and consequences of stockout events. The costly symptoms of stockout events (e.g., loss of potential sales) can manifest even in the absence of the underlying condition (e.g., an actual stockout). Lokad’s platform can be used to model all the critical subtleties where the quality of service cannot be inferred through a naïve inspection of the stock level.
4.7 Do you identify expected out-of-stock products and alert users?
Executive summary: Yes, Lokad’s platform uses advanced demand forecasting to identify potential out-of-stock situations and suggests corrective action(s) instead of just issuing alerts. These actions include expediting orders, using alternative suppliers, adjusting promotions, price points, promoting substitutes, and organizing inventory transfers (etc.).
Lokad’s platform features a state-of-the-art demand forecasting technology that can be used to identify upcoming out-of-stock events. It is also straightforward to use Lokad‘s platform to produce stock alerts for the supply chain practitioners. However, we do not recommend using alerts, as this approach does not make good use of time, particularly given timely responses are often critical. Instead, we recommend using Lokad’s platform to identify upcoming out-of-stock events and propose proactive corrective action(s).
Depending on the vertical, corrective action(s) may include (a) recontacting suppliers to expedite pending purchase orders; (b) passing complementary purchase orders from alternative (closer) suppliers; (c) cancelling upcoming promotions on items that are about to become out-of-stocks; (d) raising the price point of the items about to become out-of-stock; (e) promoting alternative products, deemed relevant substitutes, ahead of time to leverage a cannibalization effect; (f) organizing inventory transfers to reallocate sleeping inventory. These are just some of the corrective actions Lokad’s platform can promptly recommend.
Typical enterprise software (such as ERPs) still features alerts—a design pattern that invariably leads to low employee productivity. If there is something to be done about the upcoming stockout, then the software should directly recommend the action(s) as part of its normal batch of recommendations. If there is nothing to be done about the upcoming stockout, then the alert is a pointless distraction. Historically, enterprise software have used alerts as powerful mechanisms to deflect blame for poor results for end-users. This approach is—effectively—an attempt to insulate the enterprise software vendor from criticism; if the recommended inventory decision was good, then the software has performed admirably; if the recommended inventory decision was poor, then the software is blameless on account of the alert it issued. This, by definition, shifts the “blame” to the end-user. Lokad, on the contrary, takes full responsibility for the quality of our inventory recommendations and suggested corrective action(s).
See No1 at the SKU-level in the M5 forecasting competition for more on how Lokad’s approach to forecasting in practice.
5. Complications
5.1 Do you propose stock parameters for new products?
Executive summary: Yes, Lokad’s platform automates inventory decisions for new products using probabilistic, attribute-based demand forecasts, analyzing data from previously launched products. This approach focuses on direct decisions like order quantities and allocations, rather than traditional stock parameters. Lokad also considers the impact of new products on existing inventory, helping to avoid potential dead stock events. Lokad supports both demand forecasting and stochastic optimization for initial inventory orders, taking into account cross-SKU constraints and logistical capacities.
Lokad’s platform automates the entire inventory decision-making processes for new products. This encompasses the ‘stock parameters’, although we typically do not approach the challenge from this angle. New products benefit from probabilistic attribute-based demand forecasts. This is where Lokad analyzes all the previously launched products and how they fared depending on their launch settings (e.g., promotions and assortments), in addition to intrinsic qualities of the product itself (e.g., size, color, and price). Hence, the forecast for new products is automated, just like the forecast of the older products.
Furthermore, when considering new products, we consider the stock readily available to start serving the new products, and the stock that is yet to arrive. We also consider the impact on the existing stocks, and the potential negative consequences of introducing (too early) a new, possibly more attractive alternative—something that would potentially cause an immediate dead stock situation for another product.
Instead of typical ‘stock parameters’, Lokad strongly recommends approaching the challenge through direct and financially-optimized decisions. These decisions include the quantities to be ordered in the first place, and then the quantities to be allocated (SKU by SKU) for all locations. Cross-SKU or cross-product concerns must be addressed at this stage to make the most of the client’s finite logistical capacity.
These capacities are frequently insufficient to cope with the potential—and often surprising— effects of novelty. However, by spreading the workload over time, typically by effectively anticipating the launch of new products, the problem is addressed without needlessly stretching the logistical capacity of the client.
Lokad’s platform provides all the necessary numerical instruments to support this process, including the probabilistic demand forecasts for the new products and the stochastic optimization for the initial inventory orders and allocations. This support covers all the relevant cross-SKU constraints.
5.2 Can you handle the allocation of inventory during promotional phases (supporting the promotional activity)?
Executive summary: Yes, Lokad’s platform is designed to manage inventory allocation during promotional phases by aligning stock with expected future demand surges. This process involves anticipating promotional demand, integrating data from various sources like ERP systems and marketing spreadsheets, and refining predictive models to account for diverse promotional strategies. Lokad’s Supply Chain Scientists implement these models, considering not just the promotion’s immediate effects but also the risk of overstock and logistical constraints. The platform uses stochastic optimization to make risk-adjusted inventory decisions, balancing the need for sufficient promotional stock with the risk of excess inventory post-promotion.
Lokad’s general guiding principle for the allocation of the stock is to align those allocations with the expected future demand. In particular, an expected surge in future demand, as is the case for an upcoming promotion, generally requires a corresponding surge of inventory allocation. Moreover, the allocation must typically happen well ahead of the promotional event, as we need to consider the logistic constraints. For example, the network may not be able to cope with the surge of inventory movements if all the inventory is moved at the last moment. Lokad’s platform has been engineered to support this entire class of problem and all its variants.
Lokad’s Supply Chain Scientists (SCS) are responsible for the implementation of the numerical recipes that ensure the inventory allocations adequately reflect the planned promotional activities.
First, this responsibility includes gathering/organizing the data describing the promotion plans. These data are rarely found in a structured form within the business systems (ERP)—they are frequently only found in spreadsheets maintained by the marketing department. Lokad’s platform is designed to integrate multiple sources of information, including such classes of ad-hoc spreadsheets.
Second, an SCS’ responsibility covers refining the predictive model for the future demand to reflect the diversity of promotional mechanisms. Fundamentally, promotions are not just about lowering prices. They typically feature putting forward the products through promotional display (e.g., gondolas) or other communication media (e.g., newsletters). Furthermore, some mechanisms reflect cross-product mechanisms (e.g., buy one product, get 50% off another product) that must be addressed as well. Lokad platform’s features extensive predictive modeling capabilities to support all of the above.
Third, SCS’ are also in charge of generating risk-adjusted decisions that allocate sufficient inventory to satisfy promotional demand while reducing the risk of overstock after the promotion ends. When the logistical constraints are not too tight, we also consider the opportunity to leverage in-promotion replenishments to mitigate the client’s exposure to initial over-allocation risks (if the promotion is less successful than anticipated). Once again, Lokad’s platform features extensive stochastic optimization capabilities to compute these complex risk-adjusted decisions.
5.3 How do you manage, view, and distinguish different types of promotions/rewards for loyalty cardholders, including brochures, percentage discounts, monetary discounts, and exclusive promotions?
Lokad’s platform has extensive modeling support for all the pricing and promotional mechanisms found in retail. Our platform is programmatic, meaning it can be tuned to reflect any number of promotional mechanisms—we have so far identified more than 50 different examples. As our platform is also capable of processing arbitrary relational data, we can import this information exactly as it originally appears in the underlying transactional business systems.
This allows us to preserve the original data semantics, instead of forcibly reformatting the data against some vendor-defined template. Furthermore, our platform features programmatic capabilities for its predictive/machine learning capabilities as well. Through these capabilities, our Supply Chain Scientists can craft predictive demand models that reflect the mechanisms at play in the client’s promotional/reward offering(s).
Note: Lokad is only concerned with the analytical part of this problem; identifying all the patterns that shape the demand associated with a client’s promotional mechanisms. Lokad is not intended to manage promotions, e.g., in collaboration with the client’s marketing department. Though theoretically possible, it is better to maintain separation between the transactional and analytical software layers. This is See also #promotions
See also Differentiable Programming for more on the mathematical fine print surrounding our tech.
See also Complications 5.2 in this FAQ.
5.4 Do you manage UoM (unit of measure) conversion?
Yes, Lokad’s platform fully supports all UoM conversions. We also support operating with (multiple) inconsistent UoMs, as sometimes happens when Lokad processes data from different business systems. We typically resolve any UoM incompatibilities during the data preparation phase. We can also optimize inventory while constraints are expressed through distinct UoMs. For example, a Full Truck Load (FTL) comes with both volume and weight capacities.
Note: For the special case of the conversion between currencies, Lokad also has built-in capabilities, such as the forex
function that encompasses dozens of widely used currencies. This forex
function offers the possibility to apply past currency conversions in order to reflect the economic drivers as they were in the past. This allows Lokad to further optimize decision-making by analyzing the true economic impact of historical currency fluctuations, and thus further refine the client’s numerical recipe.
5.5 Do you manage Kit conversion(s)?
Executive summary: Yes, Lokad supports kit conversions and inventory optimization, including proactive kit assembly and stock reservation. Our demand forecasts account for kit elements sold separately or in multiple kits, considering varying kit life-cycles. Lokad’s financial optimization reflects the true cost of stock-outs, acknowledging the value and gross margin impact of kit elements critical to multiple high-value kits.
Lokad’s platform supports kit conversions and also supports optimizing inventory in the presence of kits. Kits are usually a simplified version of the BOM (bills of materials), and they are routinely encountered in retail business (both online and offline). The inventory optimization carried out by Lokad in presence of kits includes the capacity to decide when to reserve stock units for kits, and when to proactively assemble the kits—if the kitting process has its own capacity limit.
Furthermore, the demand forecasts generated by Lokad properly factors the reality that kit elements might also be sold/serviced separately, not just as part of a kit. These forecasts also support the case where the same kit elements are shared across several kits—naturally, we also support scenarios where kits do not share the same life-cycle, with kits entering and leaving the client’s offering with varying timelines. All these factors are properly reflected in our quantitative assessment of the upcoming need for kit elements.
Finally, when it comes to optimization of the inventory decisions in presence of kits, the financial perspective recommended by Lokad properly reflects the dependencies that exist between the kit elements and the kits themselves. For example, an element might be cheap and sold with very little gross margin, but if this element is needed by several kits that happen to have a much higher value and gross margin, then an eventual stock-out of this kit element would prove much more costly that its own value would suggest. This is because the element’s indirect value might be significantly higher than its immediately obvious direct one.
Thus, Lokad reflects through its optimization the true cost (factoring kit dependencies) of not being able to service kits due to the stock-out of one of their elements.
5.6 Do you factor an item’s code life (or shelf life) into the ordering decision and the remaining code life of products already in the supply chain?
Executive summary: Yes, Lokad’s platform achieves this by tracking each stock unit’s lifecycle, including those about to be ordered. Its core design includes scalability, efficiency in processing unit-level data, handling relational data to account for product-specific lifecycle nuances, and probabilistic forecasting to address uncertainties in product lifecycles and customer behaviors. This approach optimizes purchasing orders, stock allocations, and pricing strategies.
Lokad’s platform has been engineered to be able to track every single stock unit over time, reflecting its own specific lifecycle. Similarly, the same pattern is also applied for potential stock units, such as the ones about to be ordered. By adopting this highly granular analysis, the purchasing orders, stock allocations, and price discounts optimized by Lokad reflect the specific lifecycle(s) of every single unit flowing through the network.
Lokad’s platform makes this possible through several key aspects of its core design:
First, our platform is not only highly scalable, but highly efficient as well. Modeling flow, unit-by-unit, is more intensive than modeling it at the SKU (stock keeping unit) level; however, if the challenge is addressed by merely throwing tons of compute resources at it, then the resolution will prove to be very costly for the client company.
Second, Lokad’s platform has programmatic capabilities geared toward processing relational data. The fine-print of the life-cycle(s) of the product(s) varies a lot from one product to another. Produce and chemicals both have a shelf-life, but their respective fine-prints are very different. Lokad embraces these specificities in order to craft a model that genuinely reflects what is going on within the client’s supply chain..
Third, Lokad’s platform has general probabilistic forecasting capabilities. The life-cycle(s) of the products may come with their own batch of uncertainties. For example, in retail stores, customers may prioritize picking items with the longest remaining shelf-life. Assuming that the flow abides to a strict FIFO (first-in, first-out) behavior would be severely misguided. The predictive capabilities of Lokad’s platform are used to properly forecast/predict these subtle but immensely consequential behaviors.
5.7 Do you identify expected inventory expirations? Do you provide a shelf-life warning/alert report?
Executive summary: Yes, Lokad’s platform tracks the entire lifecycle of inventory, even without serial number tracking, and can even deploy probabilistic modeling for ambiguous situations like B2C retail. It can identify potential expirations and depreciations, issuing alerts if desired. However, Lokad prefers proactive supply chain decisions to avoid these problems, recommending actions like discounting or reallocating stock to manage items at risk of expiration.
Lokad’s platform is capable of tracking the specific lifecycle(s) of every single unit flowing through the client’s supply chain network. Our platform is capable of doing this even if units are not tracked at the S/N (serial number) level. When there is ambiguity regarding the service or consumption order of the units, as can be the case in B2C (business to consumer) retail stores, Lokad then leverages probabilistic modeling to reflect the probable states of the inventory. This fine-grained information about the state of the inventory can be used to identify probable expirations and/or depreciations and react accordingly, possibly issuing alerts directed at the relevant teams (if desired).
However, rather than alerts, Lokad recommends leveraging the fine-grained information that we have about the probable state of the inventory—down to and including the expected expiration date of every unit in stock—to proactively adjust any recommended supply chain decision (computed by Lokad). For example, we may recommend discounting or promoting a product that is at risk of suffering expirations. Alternatively, we may recommend triggering a liquidation of the products through a secondary sales channel (also at a discounted price). Another option is allocating more stock, as we acknowledge that the current stock will be soon gone, not due to customer demand, but due to expiration.
Fundamentally, if there is something that can be done about the identified potential expiration, Lokad assumes responsibility for presenting this call-to-action. Conversely, if there is nothing to be done (e.g., the stock will unfortunately expire and there is no longer any corrective measure available), then those alerts are only going to be a distraction to the teams who will not be able to do anything about it.
There is nothing easier for an enterprise software vendor than producing dozens (or even thousands) of alerts, and for many incompetent vendors, it is the only thing they know how to do. The real challenge is to produce calls-to-action, something which requires vendors (Lokad, in this case) to sort out what is actionable from what is not.
See also Complications 5.6 in this FAQ.
5.8 Can you optimize EOQ (Economic Order Quantity) considering order costs, inbound costs, inventory storage and capital binding costs?
Executive summary: Yes, Lokad’s platform can optimize EOQs considering various costs such as order, shipping, carrying, working capital, and opportunity costs—and several less obvious ones. We use a more advanced approach than the outdated Wilson formula, which is ineffective due to its oversimplified assumptions and inability to address inventory write-off risks. Lokad recommends integrating economic drivers into each purchase decision rather than focusing solely on order quantity.
Lokad’s platform has been engineered for the economic optimization of supply chain decisions. In particular, it is straightforward to reflect all the overheads associated with a purchase order, including order costs, shipping costs, carrying costs, cost of money (working capital) and opportunity costs. Furthermore, Lokad’s programmatic capabilities also make it straightforward to address all the specialized costs that may be relevant to the client’s business beyond the shortlist given above. However, we recommend internalizing the economic drivers into every single purchase decision, as opposed to singling out a quantity to be ordered, as is done in the EOQ.
The classic supply chain theory proposes to use the Wilson formula for the EOQ (economic order quantity). This approach has a severe, immediate defect: it enforces a gross rounding that proves ineffective most of time. By design, the EOQ cannot address the risk of inventory write-off. Thus, while it may be somewhat inefficient to order a quantity below the theoretical EOQ, it is, in practice, frequently a much better option as opposed to ordering more and instantly generating a sizeable write-off.
See also Inventory Costs for more on Lokad’s take regarding assessing, categorizing, and optimizing inventory costs.
5.9 Do you have KPIs for inventory in “No Conformity” status?
Yes, Lokad’s platform can easily provide “No Conformity” dashboard and/or KPIs. As our platform is programmatic, any data that can be extracted from the transactional business system can be displayed. Tis also means there is no limitation on the calculation/presentation of the KPIs—they can be completely crafted to the client’s specifications/rules. A programmatic platform is necessary here because there is no standard definition for the lack of conformity across companies.
Lokad’s platform is also capable of producing a predictive model of these non-conformity events by leveraging historical data. For example, some suppliers might have quality issues, and a fraction of their shipment may not always pass inspection. As a result, the quantities effectively available to service the client’s customers might be frequently less than those originally ordered. By modeling these occurrences with a probabilistic forecasting model, Lokad can produce risk-adjusted ordering decisions that also take this uncertainty into account.
5.10 Do you provide a report on critical materials?
Yes, Lokad’s platform can easily generate a report on whatever materials are deemed ‘critical’ by the client.
For Lokad, this is a fuzzy concept as there is no fixed definition of what constitutes a ‘critical material report’. In our experience, this varies from vertical to vertical. For FMCGs (fast-moving consumer goods), the ‘critical materials’ are typically the ones rotating the most, with the highest volumes both in eaches and in monetary units. For aviation, ‘criticality’ refers to the parts that may cause an AOG (aircraft on ground) incident if they are out-of-stock. For general merchandize stores, ‘criticality’ frequently refers to products that customers typically expect to find in the store.
Lokad’s programmatic capabilities are essential for addressing this class of requirement(s). Through these capabilities, any rule that could be implemented in a spreadsheet or in a business intelligence tool can also be implemented through our platform. Not having such programmatic capabilities would force supply chain practitioners to revert to spreadsheets as they cannot afford a vague approximation of whatever is deemed ‘critical’ by their company. The analytical layer (Lokad in this case) must be able to fully adhere to the intricacies of the business, hence the need for complete programmatic functionality and freedom.
6. Reporting
6.1 Do you have KPIs for the total amount of inventory? Specifically, do these cover turnover and coverage rates? Additionally, can these KPIs be segmented according to part number (P/N), product platform, and product quality, as well as provide a monthly progress report across different activities and sectors based on the quality of the products?
Executive summary: Yes, Lokad offers extensive reporting capabilities, including customizable KPIs for inventory management, adaptable to client-specific requirements like part number, product platform, and product quality. Our domain-specific language (Envision) simplifies data visualization and dashboard creation, addressing the unique data structures of each company without the need for standard data formats, thus avoiding lengthy integration projects common with traditional enterprise software. This approach ensures high productivity, reliability, and scalability in generating bespoke reports and managing supply chain optimization.
Lokad’s platform features extensive built-in reporting capabilities. As a rule of thumb, any report that can be produced with a spreadsheet or with a business intelligence tool can also be produced by Lokad. Lokad has engineered a DSL (domain-specific programming language) dedicated to the predictive optimization of supply chain (named ‘Envision’). As the name Envision suggests, this DSL puts a major emphasis on facilitating the visualization of data. Lokad’s Supply Chain Scientists are typically responsible for the setup of all relevant dashboards and KPIs. Moreover, Lokad’s platform has been engineered to support constant-time rendering of complex dashboards. This allows us to craft dashboards that gather all the relevant items in one place, alleviating the need for supply chain practitioners to navigate a maze of disconnected screens/displays while trying to get the information they need for their daily routine.
All the reports produced by Lokad are “bespoke” for the client company—unlike what one typically finds in similar software. The reality is producing such reports is only possible using a flexible, programmatic platform, given the reports—even “basic” ones—are entirely dependent on the unique fine-print of the client in question (including their applicative landscape). In our experience, no two companies are the same, even if they use the same software to organize their business data in the exact same way.
In a production-grade setting, mundane “data plumbing” represents over 90% of the work invested in generating such reports. Thus, through Envision, Lokad frontally addresses the productivity challenge associated with this data plumbing. Moreover, this approach gives us the possibility to preserve all the pre-existing codifications, hierarchies, and conventions in the client’s other business system(s). Hence, Lokad ends up “speaking the exact same language” as the client’s staff, instead of introducing yet another set of conventions that employees must figure out.
The mainstream approach in enterprise software consists of establishing a set of data requirements. Once the input data matches those requirements, all the reporting and analytical features are unlocked. Unfortunately, this approach works poorly for anything but the smallest companies. There is never a one-to-one matching between the original business systems (the source of the data) and the packaged analytical systems. As a result, the translation of the data is both incredibly demanding and frustrating as it is simply not possible to get everything to fit. What seemed to be a simple matter of “configuration” to import the business data into the packaged analytical system invariably turns into a year-long integration project. This is the inescapable consequence of attempting to reconcile two distinct complex perspectives on supply chain. Lokad’s platform design choices eliminate this problem entirely.
6.2 Do you have inventory health and coverage reports?
Executive summary: Yes, Lokad provides inventory health and coverage reports through advanced probabilistic forecasts and tools on its platform, allowing precise risk assessment(s)—typically expressed in monetary terms. These financial risk assessments are the fundamental “health risks” to the client’s inventory. Unlike traditional time-series forecasts which consider only one future, Lokad’s approach accounts for multiple possible future values (e.g., demand), offering a more accurate and comprehensive view of inventory risks, thus helping to avoid—or at least reduce—inventory health issues.
Lokad’s default practice is to provide inventory supervision instruments, such as inventory health and coverage reports. Lokad’s Supply Chain Scientists are responsible for generating these reports. Lokad’s platform comes with extensive, programmatic reporting capabilities. This includes all the necessary tools to convert probabilistic forecasts—regarding demand, lead time, and all relevant sources of uncertainty—into high-level projections, ideally reflecting dollars of inventory costs, as opposed to percentages.
The ‘health’ of the inventory is always relative to the future expected conditions of the market. The number of units in stock is only considered excessive if it is well beyond the expected future demand, not if it is well beyond the past observed demand. E.g., past demand might be zero for a new product or for a product that suffered an enduring shortage. Thus, the crux of the challenge is to turn the projections/forecasts into reports.
Here, probabilistic forecasts (favored by Lokad), which consider all possible futures and assign them probabilities, are ideal. This is because they allow us to precisely assess the inventory risks by assigning probabilities to the likelihood of a risk, and subsequently evaluating the potential financial impact associated with that risk. It is these risks, expressed in monetary terms (e.g., dollars or euros) that are reflected in the reports Lokad provides.
In contrast, classic time series forecasts—by design—only consider one possible future value (e.g., demand). As such, these forecasts are not capable to adequately convey the wide array of financial risks the client may encounter based on their inventory decisions. The sophistication of time series forecasts is irrelevant: the form/structure of the forecast does not convey the required information. Some attempts to evade this problem involved crude heuristics to assess the inventory risks through a direct inspection of the recent past. One example included counting the SKUs that have over X weeks’ worth of inventory on hand (e.g., 2 or 3). However, these methods invariably produce low-quality indicators that mislead supply chain practitioners rather than enlighten them.
6.3 Can you alert the procurement team when a PO (Purchase Order) needs to be postponed or expedited?
Executive summary: Yes, Lokad can issue these “alerts”, though we prefer the term “decisions”. Lokad’s platform generates an automated list of decision-making recommendations, including reactively revising purchase orders (POs) based on economic benefits and supplier cooperation. We prefer “decisions” to “alerts”, as our recommendations are direct calls to action (with modelized costs and benefits), rather than simply informing the client of a potential problem.
Lokad automates the generation of decisions with a perspective that includes postponing or expediting POs as needed. These decisions are prioritized in terms of economic reward (much like typical Lokad decisions), and the drivers include the economic benefits associated with a revised PO. These drivers may include may include negative goodwill to reflect the overhead generated for the supplier, and the likelihood of getting the revision accepted and implemented by the supplier. Similarly, Lokad can suggest revising the PO quantity up or down—assuming the supplier is amendable to this option. Lokad’s Supply Chain Scientists establish the fine-print of the options available as far as the POs are concerned and then automate the logic that generates the corresponding “alerts”—something Lokad would rather call “decisions”.
Lokad refers to “alerts” as “decisions” because each one of the recommendations has potential costs and benefits, leading to tangible consequences for the supply chain. In this regard, the recommendations are not fundamentally different from recommending placing a PO in the first place. The main difference is the uncertain willingness (or capacity) of the supplier to accommodate the requested correction for the PO, but in the case where the supplier complies with the request, this recommendation is as much an “order” as the original PO.
Many enterprise software offer an old (now obsolete) perspective on supply chain decisions, limiting such decisions to narrow textbook situations such as purchasing or rebalancing stock. This type of software ignores, by design, all nuanced options that may be on the table with the suppliers. E.g., the supplier may have the possibility to expedite, postpone, inflate or deflate its order, and even possibly substitute some products for others. Sometimes, the supplier has the possibility to send a fraction of the PO early, if the client company is willing to support the overhead for multiple shipments. Sometimes, untouched goods can be returned to the supplier for a specific period of time. The programmatic capabilities of Lokad’s platform are a necessary technological ingredient to address these nuanced options.
6.4 Can you alert the procurement team when an expected delivery date is reached/overdue?
Yes, Lokad’s platform can easily generate and issue alerts when an expected delivery date becomes overdue. Our probabilistic forecasting approach allows us to refine these alerts in the presence of varying lead times.
A probabilistic forecast of lead time can be used to assess whether a given delay is genuinely anomalous or just part of the everyday inconsequential variations observed in supply chain. Furthermore, alerts can be prioritized to reflect the expected economic impact of the delay. For example, if the demand has unexpectedly dropped, the extra lead time may accidentally turn out to be inconsequential, and thus does not warrant the immediate attention of the procurement team. Fundamentally, every moving part of the supply chain competes for the attention of the procurement team. This attention is a scarce resource, and is ultimately one that Lokad aims to maximize with the alerts it generates.
Fundamentally, our take is that issuing “alerts” (as popularly understood) is an obsolete approach, indicative of poorly designed enterprise software. “Alerts” typically call the client’s attention to an issue, rather than providing an actionable recommendation/decision based on a clear financial assessment of the situation. Lokad believes it is essentially our responsibility to generate risk-adjusted decisions for clients, instead of distracting them with basic alerts. For this reason, though we do issue them, our use of “alerts” should be understood to mean “profitable corrective actions/measures in response to a problem”.
See also Reporting 6.3 in this FAQ.
7. Productivity
7.1 Can users manually define replenishment thresholds and/or manually override stock parameters?
Executive summary: Yes, Lokad’s platform allows users to manually set replenishment thresholds and override stock parameters, including adding temporary adjustments. However, we strongly advise against frequent manual overrides. Instead, if there is an issue with the decisions Lokad generates, we would rather update/refine the underlying numerical recipe (algorithm) that generates them. Lokad—philosophically, technologically, and methodologically—aims to surpass dated and unreliable approaches that rely on manual interventions, preferring reliable and scalable automation.
Lokad’s platform is highly configurable, thus the workflow for inventory replenishment can easily include manual data entries/overrides for the replenishment thresholds or other similar stock parameters (e.g., min/max, safety stock, etc.). Moreover, it is also possible to consider variants such as assorting the manual data entries with ‘expiration date(s)’ if the supply chain practitioners expect the situation to revert to normal in a few weeks or months. This would let the regular numerical recipe regain control of the replenishment process. While a hard-set threshold might be beneficial in the short term, it is almost invariably a problem in the mid-term, as the threshold loses its original relevance.
More generally, Lokad strongly advocates not relying on manual overrides to govern mundane inventory replenishments. If the numerical recipe that governs the inventory replenishments has issues, then this recipe must be urgently fixed. Lokad’s Supply Chain Scientists are trained to deliver necessary fixes in a timely manner. Choosing instead to rely on manual overrides is tantamount to having client staff duct-tape short-term fixes. This is clearly inefficient, and contrary to one of the central consequences of Lokad’s Quantitative Supply Chain theory, namely redirecting time, resources, and bandwidth to higher-value tasks.
Lokad’s approach stands in direct opposition to many enterprise software vendors who provide obsolete technologies that lock the consumer into making regular—inefficient—overrides. This dynamic is predicated on a tacit understanding that the customer is responsible for correcting the garbage the software produces. Responsibility is often deflected from the software vendor by issuing “alerts” that draw the client’s attention to a suboptimal situation (without providing useful corrective action). As a result, whenever the replenishment is wrong, the blame is deflected to the practitioner who should have manually overridden the system. Lokad believes this to be an outrageous practice, precisely why we do not do it.
7.2 Can you automate stock parameters based on pre-defined or user-defined variables (e.g., supplier performance, target service level(s), demand variability, SKU class, shelf life, etc.)?
Executive summary: Yes, Lokad automates inventory decisions, including stock parameters, through a fully automated daily process with minimal manual intervention. This automation adapts to large disruptions as needed. The process leverages probabilistic forecasts to address uncertainties like varying demand and lead times, and a robust financial approach (implemented through stochastic optimization) that considers the totality of costs and constraints. This allows Lokad to maximize service and minimize financial errors in the presence of uncertain supply chain conditions.
Lokad’s default approach is to automate the entire process that governs the computation of inventory decisions, such as replenishment quantities. For Lokad, the norm is to have a fully automated daily process that does not require any manual intervention. The vast majority of our clients operate for weeks without any manual intervention. Naturally, when unprecedented large disruptions happen (e.g., systemic shocks like lockdowns), Lokad’s Supply Chain Scientists are ready to step in and adjust the numerical recipe(s) to mitigate the disruption. However, we believe mundane, routine decisions must be fully automated, so as to redirect time and mental effort to higher-order tasks (such as business strategy).
The automation of the update of all the stock parameters happens at two levels: the predictive model and the stochastic optimization.
Lokad uses probabilistic forecasts for all relevant sources of uncertainty, such as lead times, returns, etc. For example, in the lockdown scenario mentioned above, the supplier performance case is implicitly a lead-time variability problem that must be addressed through probabilistic lead time forecasts. Lokad’s platform is not only capable of producing all those probabilistic forecasts, but also of combining the forecasts into a unified probabilistic view of the future of the company. Within Lokad’s platform, we would typically leverage differentiable programming and our algebra of random variables for this purpose.
When it comes to the optimization itself, we must consider all the relevant costs and constraints. For example, in the same lockdown scenario, shelf life would implicitly refer to a specific type of nonlinear carrying cost. One of the reasons why Lokad promotes a financial approach to inventory optimization is that it facilitates the blending of many disparate-seeming concerns into a unified numerical perspective. This numerical perspective helps Lokad to minimize the dollars (or euros) of inventory error, while maximizing the dollars of return obtained by properly servicing clients. With Lokad’s platform, we would typically leverage our general stochastic optimization capabilities for this purpose. The ‘stochastic’ part refers to Lokad’s capacity to perform an optimization under noisy/uncertain/random conditions.
7.3 Do you automate PO (Purchase Order) issuance?
Executive summary: Yes, Lokad automates purchase order (PO) generation, relying on an automated data pipeline for input from (and output to) business systems, ensuring up-to-date and synchronized orders. It functions as an analytical layer on top of transactional systems, calculating optimized quantities but not executing transactional steps like PDF creation. Lokad also offers a semi-automated option with partial validation workflows, allowing manual oversight for critical orders while automating routine ones.
Lokad automates the generation of optimized purchase orders. This automation requires an automated data extraction pipeline between the client and the Lokad platform. This data pipeline ideally traffics daily updates, ensuring that Lokad operates with fresh data. This keeps the generated purchase orders in sync with the state of the business. This automation also requires an automated data export pipeline from Lokad toward the original business systems, where the mundane, purely transactional steps of the ordering process can be completed.
Lokad is not a replacement for a transactional business system, such as an ERP. Lokad is an analytical layer that operates on top of the transactional business system. Lokad will compute the optimized quantities (i.e., how much should be ordered and when) for every purchase order. However, Lokad will not generate a PDF order file to be sent by email to the supplier (for example). These steps belong to the realm of the transactional business systems. For this reason, Lokad generates flat tabular files that contain all the necessary information. This data is subsequently exported to the client’s business system for execution.
It is possible for us to establish a semi-automated process with a partial validation workflow within Lokad’s platform. For example, the client company might decide that “trivial” purchase orders get automatically validated (typically the small ones), while requesting a supply chain practitioner to step in and manually validate the recommended order quantities suggested by Lokad above a certain threshold/desired parameter. These rules can be updated over time as well. As the project continues, and clients see the value generated, the threshold for manual validation tends to be increased. Ultimately, this alleviates the burden on the client’s supply chain team(s).
7.4 Do you have a planner/buyer window that displays the set of tasks for the day, organized by priority?
Executive summary: Yes, Lokad’s platform provides a special unified dashboard, specifically engineered each day for high-order corporate functions. This single window organizes and displays tasks, including calls-to-action like PO management and data anomaly inspections, and prioritizes them in terms of monetary impact.
When using Lokad’s platform, we recommend for every corporate function, gathering in a single web dashboard (i.e., a single window) all the calls-to-action ordered by priority. The priority should be expressed in monetary terms (dollars or euros of impact) that genuinely reflect what is at stake if the call-to-action is not processed. For a demand and supply planner, this dashboard would typically include the recommended new POs (purchase orders), as well as any old POs that require further attention (e.g., expedite, postpone, inflate, shrink). Lokad’s Supply Chain Scientists are typically responsible for arranging the dashboards in a way that aligns with each client’s unique corporate structure. This is critical for such a dashboard given boundaries between procurement/planning/inventory management/purchasing/finance teams tend to vary from one company to another.
These calls-to-action may also include inspecting data anomalies that prove consequential for the client, such as incorrect retail prices, incorrect MOQs (minimal order quantities), incorrect stock levels, etc. These calls-to-action are also prioritized with respect to their potential economic impact. More generally, Lokad avoids two pitfalls that are all too common in enterprise software. First, we do not spread the workload of a given user across dispersed screens (or windows/web pages).
On the contrary, Lokad’s platform has been specifically engineered to deliver complex dashboards in constant time. This technical detail proves critical when it comes to gathering numerous disparate elements in a single dashboard for the benefit of the end user. Second, Lokad’s platform has also been specifically engineered to support end-to-end economic analysis, hence unifying all the calls-to-action under a common prioritization logic expressed in monetary terms.
Notes
-
In simple terms, this refers to the sophisticated algorithm used to generate the client’s supply chain decisions. It is crafted to reflect the client’s particular supply chain goals, as well as to consider the totality of their constraints and drivers. ↩︎
-
The decisions recommended by Lokad are typically whiteboxed through their economic drivers. The decomposition of factors (expressed in euros or dollars of impact) explain ‘why’ a certain decision is recommended. This information is conveyed through multiple customizable dashboards. See Lokad’s Technology for more information on how clients interact with their supply chain decisions. ↩︎