Quantiles = Inventory Optimization 2.0
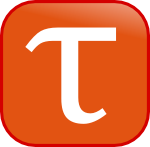
Getting more accurate forecasts, that turn into profits, is the No1 priority for Lokad. However, demand forecasting has been extensively researched for more than a half a century, and each 0.1% of extra accuracy is typically nothing less than a uphill battle.
Sometimes though, we make a breakthrough. Today, we are announcing the most significant technology upgrade of Lokad since its launch 6 years ago: the immediate availability of quantile forecasts.
Quantiles are disruptive in the sense that in many situations they make classic forecasts plain obsolete as far inventory optimization is concerned - for retail, wholesale and manufacturing businesses.
We have identified 3 situations where quantiles really shine:
- High service levels at 90% and above.
- Intermittent demand (slow mover’s).
- Bulk orders (spiky demand).
In those situations, benchmarks against our own classic forecasting technology indicate that quantile forecasts typically bring either 20% less inventory or 20% less stockouts.
Extraordinary claims require extraordinary evidence. Carl Sagan
However, the many benchmarks that we have made so far with our prospects and clients indicate that our classic forecasting technology is already ahead of the competition; but with quantile forecasts, it’s whole new level of inventory optimization that can be achieved.
Don’t hesitate to put quantiles to the test.
The story behind the quantile upgrade
Quantile forecasting (also called quantile regression) has been around for decades among academic circles. Then, in finance, analysts have been extensively using quantiles for Value at Risk (VaR) analysis since the late 1980s.
At Lokad, quantiles have been around for a long time as well. For example, back in 2009, Sequential Quantile Prediction of Time Series. IEEE Transactions on Information Theory, March 2011, vol. 57, n°3 has been published by one of us. However, until very recently, quantiles were very mistakenly considered a mathematical distraction (business-wise) rather than a mission critical concept.
What did hold us back was not lacking insights in statistics, but lacking insights in the profound relationship between quantiles and inventory optimization. This insight was triggered, mostly out of dumb luck, when a client did ask us to figure out a formula to compute optimal service levels for her inventory.
A breakthrough yes, but a late one
This quantile breakthrough is only very relative in the sense that quantiles have been already applied successfully for decades in other trades. However, there is one aspect that partially explains this late arrival: quantile models typically require about 10x more processing power compared to classic forecasting models. Without cloud computing, we would not have been able to put quantiles in production, while preserving an aggressive pricing.