Show me the money: Reflections on ISF 2024
“In order to improve your game, you must study the endgame before anything else; for, whereas the endings can be studied and mastered by themselves, the middlegame and the opening must be studied in relation to the endgame.” Source: Capablanca’s Last Chess lectures (1966), p. 23
A few weeks ago, I spoke on a panel at the 44th International Symposium on Forecasting in Dijon, France. The topic of the panel was Demand Planning and the Role of Judgement in the New World of AI/ML.
As an ambassador for Lokad, you can imagine what my perspective was:
-
forecasting and decision-making should be completely automated;
-
forecast quality should be evaluated from the perspective of better decisions;
-
human judgement should be used to improve the automation (not tweak forecasts or decisions).
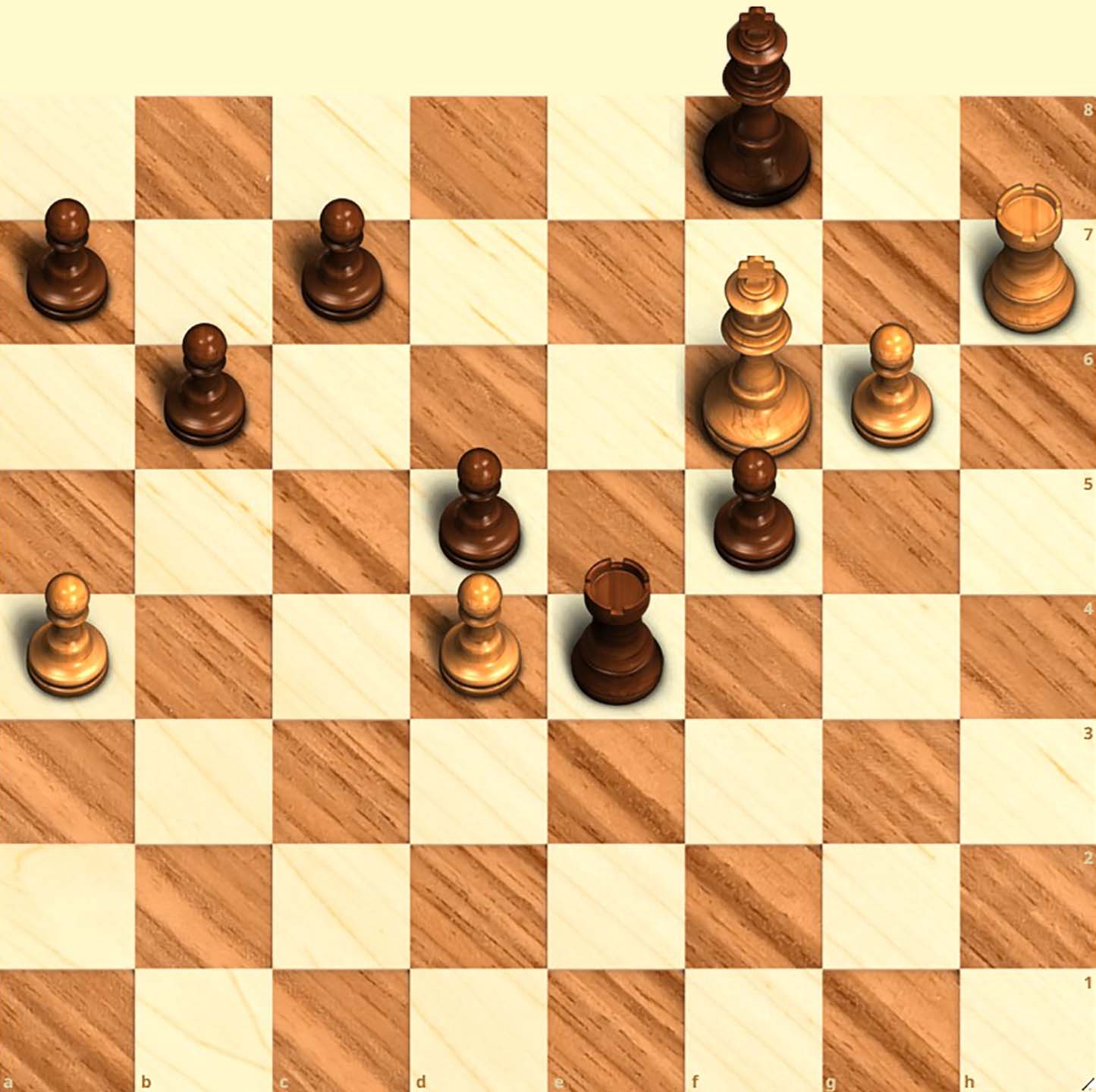
Oddly enough, my position on automation did not provoke as much disagreement as you might think. The chair (Lokad’s Head of Communication, Conor Doherty) and the other panelists (Sven Crone of iqast and Nicolas Vandeput of SupChains) were almost unanimously in agreement that this was the future of forecasting. The only disagreement was how quickly we might reach this state (note: I believe we are already there).
What did cause quite a bit of disagreement, and perhaps even confusion, was my argument that forecast accuracy is not nearly as important as making better decisions. This disagreement was not confined to the other panelists, but members of the audience, too. I think there are two major reasons for this:
-
When I spoke on stage, I did not have a visual to support this point. There are a few moving parts in the explanation, so a visual would have definitely helped people to understand.
-
The idea that forecast accuracy is less important than decisions contradicts most professionals’ education, training, and experience.
By the end of this essay, I hope to have addressed both the points listed above. Regarding the first point, I have included a short but systematic explanation and an intuitive visual. Regarding the second point, I can only ask the reader to maintain an open mind for the next 5 to 10 minutes, and try to approach these words as if you had zero previous training in supply chain forecasting.
Guiding questions
There are, in my opinion, five foundational questions that need to be answered to clarify my position. In this section, I will do my best to provide short(ish) answers to each one - the “meat and potatoes”, as it were. Rest assured, Lokad has a wealth of additional resources to explain the technicalities, which I will link to at the end of the essay.
Q1: What does it mean for a forecast to “add value'?
I will immediately start with an example. Let’s assume there is a default mechanism for producing decisions in a company (e.g., automated statistical forecast + automated inventory policy).
For a modified forecast to add value it needs to change a default decision (generated using the company’s default process) in a way that directly and positively affects the company’s financial returns (i.e., dollars, pounds, or euros of return).
If a forecast is more accurate (in terms of predicting actual demand) but does not result in a different and better decision being made, then it has not added value.
Many companies still use time series forecasting models, whereas Lokad prefers probabilistic forecasts to help generate risk-adjusted decisions. However, the same standard applies to both forecasting paradigms. For either forecast type to add value, it must alter a default decision in a way that directly and positively affects a company’s financial returns.
For example, a new (“altered”) decision might directly eliminate a future stock-out that the default decision would have presented.
“Directly” is critical here. In very simple terms, the forecast only adds value if you can point to the exact decision change that influenced the additional financial returns or prevented financial losses (in comparison to the default decision).
Think causation, not correlation.
Q2: Does a more accurate forecast always add value?
Technically, no. A more accurate forecast, in and of itself, does not necessarily “add value”. This is because, as mentioned earlier, for something (in this case a forecast) to add value it must directly and positively affect a company’s financial returns through a better decision.
Unlike forecasts, supply chain decisions have feasibility constraints (e.g., MOQs, lot multipliers, batch sizes etc.) and financial incentives (e.g., price breaks, payment terms, etc.). There can be many more forecasts than there are feasible decisions.
This means supply chain decisions can occasionally be (and very often are) insensitive to forecast accuracy changes. This is true for both time series and probabilistic forecasts.
The reason for this insensitivity is due to decision-making constraints (e.g., MOQs). It is perfectly possible that a more accurate forecast (e.g., 10% more accurate) leads to the exact same decision as a less accurate one. The chart below illustrates this point.
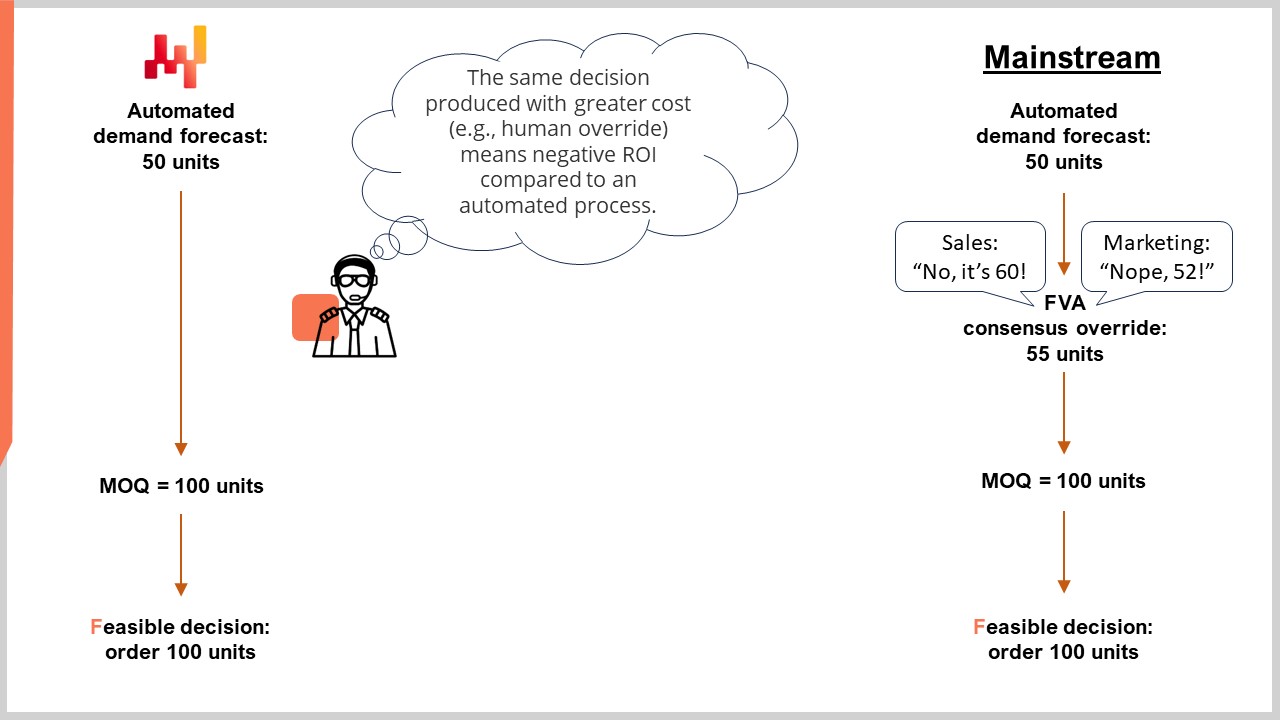
In the above example, let’s say the consensus forecast of 55 units was more accurate than the automated forecast of 50 units. From a financial perspective, the increased accuracy did not result in a different decision (due to the presence of an MOQ). Thus, the more accurate forecast did not add value.
In fact, there is a strong argument that the more accurate consensus forecast resulted in negative value added. This is because the extra review steps (as per a standard Forecast Value Added process) cost money (extra time and effort) for the company, yet did not result in a better decision. From a purely financial position, those manual review steps were a net negative.
Let’s also consider a case where there is no MOQ constraint.
Imagine the same overall scenario, but there is a lot multiplier instead of an MOQ. The feasible decisions are increments of 50 units (e.g., 50 units in a box or on a pallet). In this situation, we would have to buy either 50 or 100 units (1 or 2 boxes or pallets).
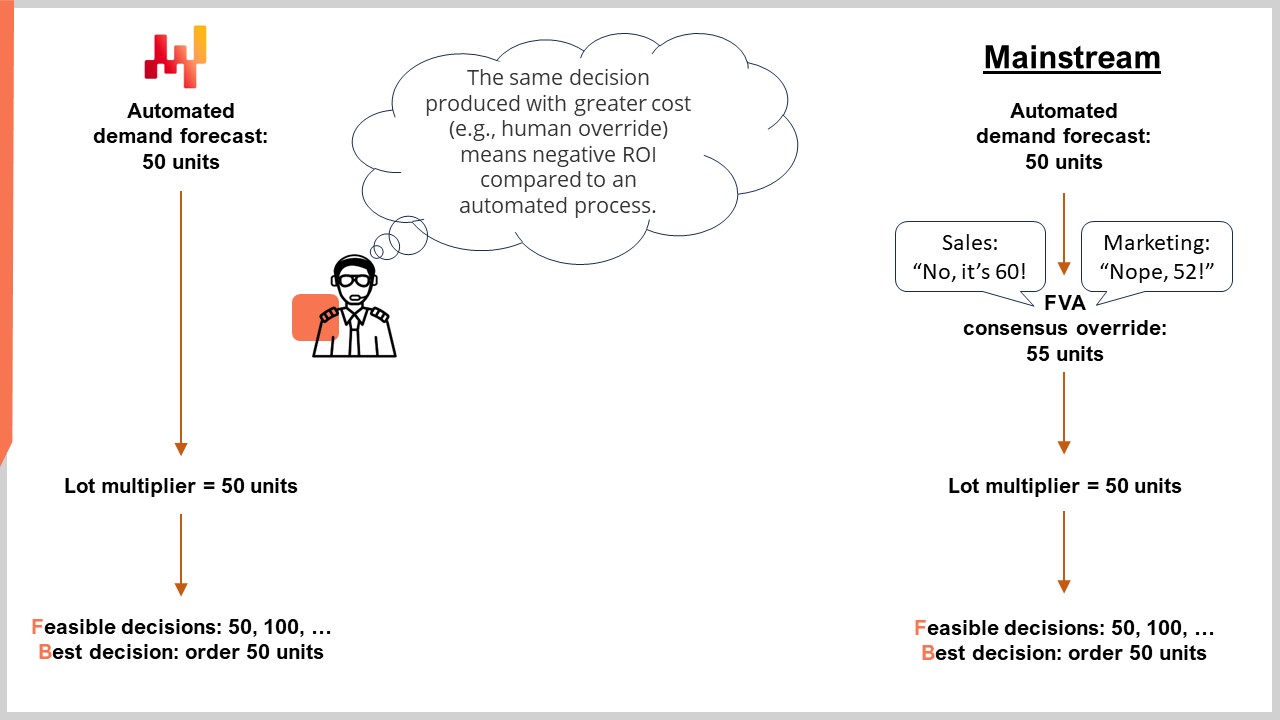
In reality, it might be less profitable to buy 100 units (covering the consensus forecast’s suggestion of 55 units) than buying 50 units (slightly less than the “more accurate” forecast suggests). One could try to cover the remaining demand with backorders or simply lose sales (e.g., if selling perishable goods like fresh food).
From an economics perspective, the best financial decision might not be to follow the “more accurate” forecast. In this scenario, both the automated (50 units of demand) and consensus forecast (55 units of demand) result in the same decision (order 50 units). Thus, the “more accurate” forecast did not result in increased financial value.
Granted, not all situations are equally strict when it comes to constraints, however supply chain is filled with such types of scenarios. Of course, I concede that different forecasts will result in producing different decisions, but the question of value remains open. At all times, we should consider if the expected additional return from purchasing extra units are greater than the extra resources consumed to improve forecast accuracy.
Maybe the extra accuracy is worth it in some situations. However, forecasters and supply chain practitioners seem to reflexively assume that it is in absolute terms, despite the fact there are obvious scenarios in which it is not.
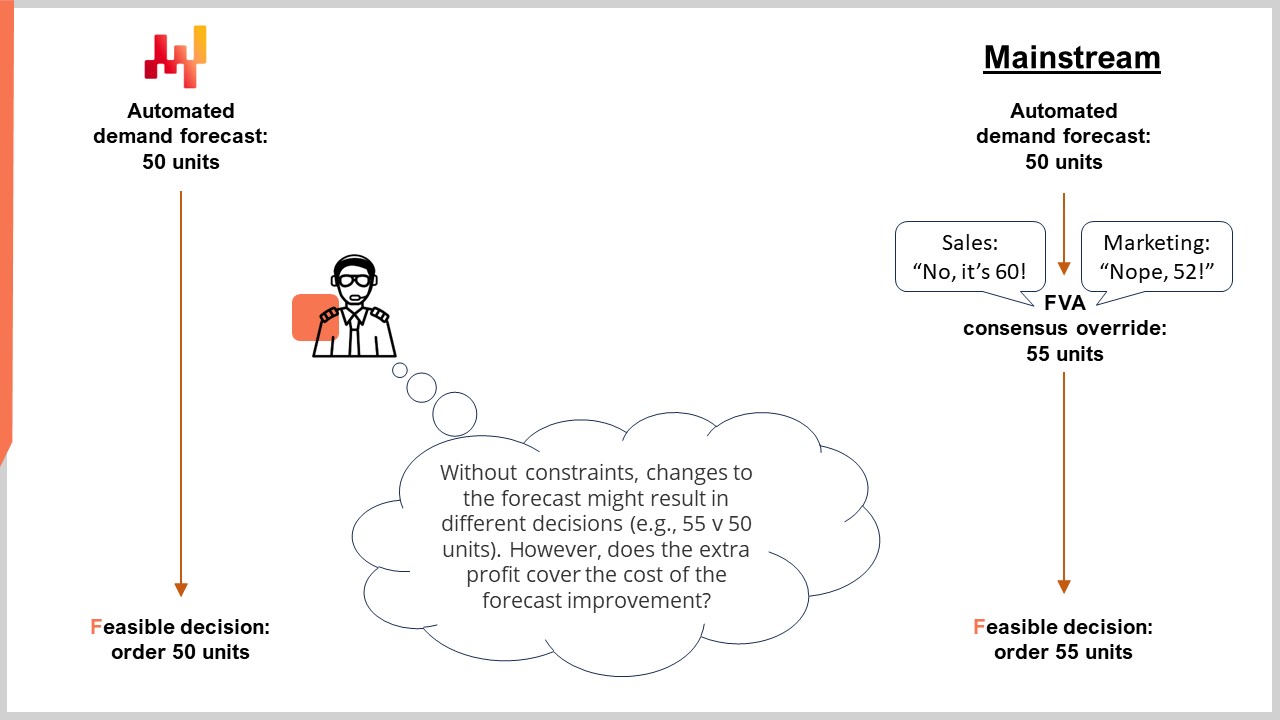
If you have thought of a scenario that does not perfectly match the examples described here, that’s fine. Remember, the goal today is to demonstrate a general point (that there are situations when extra forecast accuracy is not worth pursuing), not to analyze in depth every possible supply chain decision-making scenario.
Q3: How can we ensure that the value gained is worth the cost of judgmental intervention?
A core element of the panel discussion in Dijon was the value (or not) of judgmental intervention (or “human overrides”) in the forecasting process. To paraphrase the other side, “we have to have people in the loop to correct when the automated forecast has missed something”.
This is a very interesting perspective to me, as it presumes that human override adds value - otherwise, why on earth would anyone do it?
For this section, I’m going to ignore a discussion on whether or not humans can (occasionally or even often) outperform an automated forecast (in terms of accuracy). In fact, I’m willing to concede that, on any isolated SKU, a human can perform as well or perhaps even better than an automated forecast in terms of accuracy.
Note: I do not think this is true if we consider forecasting tens of thousands of SKUs for hundreds of stores, every single day, as per a sizeable supply chain1. In the latter scenario, an automated forecast significantly outperforms entire teams of incredibly skilled forecasters and other functional experts simply because the vast majority of SKUs cannot be manually reviewed due to time constraints.
I’m making this concession that human judgment can sometimes match or exceed automated forecasting for two reasons:
-
In my opinion, it makes the essay more interesting, and;
-
The strength of my argument does not rest on any discussion of “accuracy”.
My position is, as you can probably guess by now, that human overrides only “add value” if they…add financial value - value that lasts longer than a single reorder cycle. This is completely independent of any accuracy benefits.
This value can be understood as “directly produces better decisions than were originally generated - factoring the extra profits from the better decision and subtracting the cost of the override”.
Simply put, judgmental interventions (human overrides) are costly, thus a company should want to see a significant return on investment. It is thus my argument that forecast accuracy is an arbitrary metric (when evaluated in isolation from decisions), and companies should focus on actions that increase financial returns.
Human override may very well increase forecast accuracy (again, I make this concession for the sake of discussion), however it does not necessarily increase financial return. This really should not be a radical proposition, in the same way that someone can be both the tallest person in one room and the shortest person in another.
Please note that the responsibility is not on me to provide evidence that increased accuracy does not translate to increased profits. It is, by definition, the responsibility of people arguing that increased accuracy is in and of itself profitable who must provide some concrete, direct, and indisputable evidence for this claim.
Again, this should not be a radical or contrarian position. It should, in my opinion, be the default position of anyone “with skin in the game”.
Bear in mind, for human overrides to be profitable, we have to take the totality of overrides into consideration. This is to say, weigh up the financial value generated by all the “hits” and subtract all the financial losses inflicted by the “misses”.
This experiment would also need to be done at scale, for an enormous network of stores (enterprise clients in the case of B2B) and across their entire catalogue of SKUs, every day, for a considerable amount of time.
“How long should this experiment run, Alexey?” On this, I’m ambivalent. Let’s say a year, but I’m very open to discussion on this point. It depends on many things including the number of decision cycles in a year, as well as lead times, naturally.
That said, this entire discussion raises the question of what the acceptable threshold of error is for human override.
- If the hits slightly outweigh the misses, is that acceptable?
- What about the cost of the human overrides themselves?
- How are we to factor these direct and indirect costs into the calculus?
These are not trivial questions, by the way. They are the kinds of question a freshman student would ask in any introductory course in a STEM (or STEM-adjacent) field.
Until such time as someone provides definitive proof that human override, deployed at scale, is financially worthwhile, the most economically intelligent position is to presume that it doesn’t and to continue relying on automated forecasts and automated decision-making.
Q4: How do we determine when a more accurate forecast should replace the current forecast for decision-making purposes?
In short, the easiest way to know is to consider the following question: does the new forecast result in better decisions? The evaluating metric in this case should be financial return on investment (ROI).
To get a bit more granular, the replacement should be made based on the new model’s overall comparative utility (e.g., ROI, applicability, maintainability, etc.), not only based on its current accuracy gain. ROI is what steers the company in the direction of success. Applicability, as I’ll demonstrate below, is designed with an eye towards ROI. Remember: accuracy is, if pursued in isolation, an arbitrary KPI.
For example, imagine we have two models: one that can deal with stock-out history explicitly and another one that ignores stock-outs (using some data preprocessing tricks). It might be the case that stock-outs didn’t happen that often, and from a decision-making perspective both models perform almost equally. However, it would still be more prudent to favor the model that can deal with stock-outs. This is because, if stock-outs start to occur more frequently, this model will be more reliable.
This demonstrates another aspect of Lokad’s philosophy: correctness by design. This means, at the design level, we aim to engineer a model that proactively considers - and is capable of responding to - both likely and unlikely events. This is of paramount importance because the biggest financial penalties often lie at the extremes - the unlikely events, in other words.
Q5: How do we transition from one forecasting model to another in production?
It is important to remember that forecasting is just one part of the overall decision-making engine. As such, updating some parts may have minor or major impacts on the engine’s overall performance. Transitioning from an old model to a new one might be problematic, even if the new model will ultimately generate better decisions (thus delivering more profits).
This is because the improved decisions in theory may meet unprecedented constraints in reality if implemented too quickly.
For example, a new forecast model may help to generate much improved POs, but the space required to store the extra inventory may not exist yet or suppliers can’t immediately adjust their supply chains to satisfy the increased demand. Rushing to complete the POs now, in search of immediate profits, may result in losses elsewhere, such as stock being damaged or perishing faster due to a lack of adequate warehouse space (or workforce capacity limits).
In such a scenario, it might be wise to progressively transition between models. In practice, this might involve placing a few slightly larger consecutive POs to gradually correct stock positions, rather than immediately passing a single enormous one.
People with hands-on experience tackling the bullwhip effect (including DDMRP advocates) should immediately see why this is a wise tactic.
Closing thought
If you have read this far, I appreciate your attention. If you have disagreed along the way, I appreciate your attention even more.
For those who disagree, allow me one last swing: value means more money, and more money comes from better decisions. As far as I am concerned, nothing can substitute good (or better) decisions. Not a more accurate forecast. Not a more efficient S&OP process.
If we still disagree, fair enough, but at least we know where we both stand.
Thanks for reading.
Before you leave
Here are a few more resources that you might find useful (especially if you have disagreed with me):
-
Regarding how Lokad actually forecasts all sources of uncertainty (e.g., demand, lead times, return rates, etc.), see our video lectures on probabilistic forecasts and lead time forecasting.
-
Regarding how Lokad actually makes risk-adjusted decisions, consult our educational tutorial on purchasing optimization and video lecture on retail stock allocation.
-
Regarding how Lokad engineers demand and optimizes pricing strategies, see our video lecture on pricing optimization.
Notes
-
The largest supply chains account for even larger numbers – tens of thousands of stores in over a hundred countries with several hundred distribution centers. The catalogues of such giants often contain hundreds of thousands (if not millions) of different products. ↩︎