A Nuanced Perspective on Harvard Business School’s Jagged Technological Frontier
Quality is subjective; cost is not.
In a recent episode of LokadTV, I suggested that a paper by Harvard Business School (HBS), conducted in collaboration with Boston Consulting Group (BCG), was deeply flawed and potentially dangerous. The paper’s full title is Navigating the Jagged Technological Frontier: Field Experimental Evidence of the Effects of AI on Knowledge, Worker Productivity, and Quality1. Succinctly, the paper stated that AI’s abilities at demanding tasks is unevenly spread, as it excels in some tasks and performs poorly at others (“inside” and “outside the frontier” tasks, respectively).
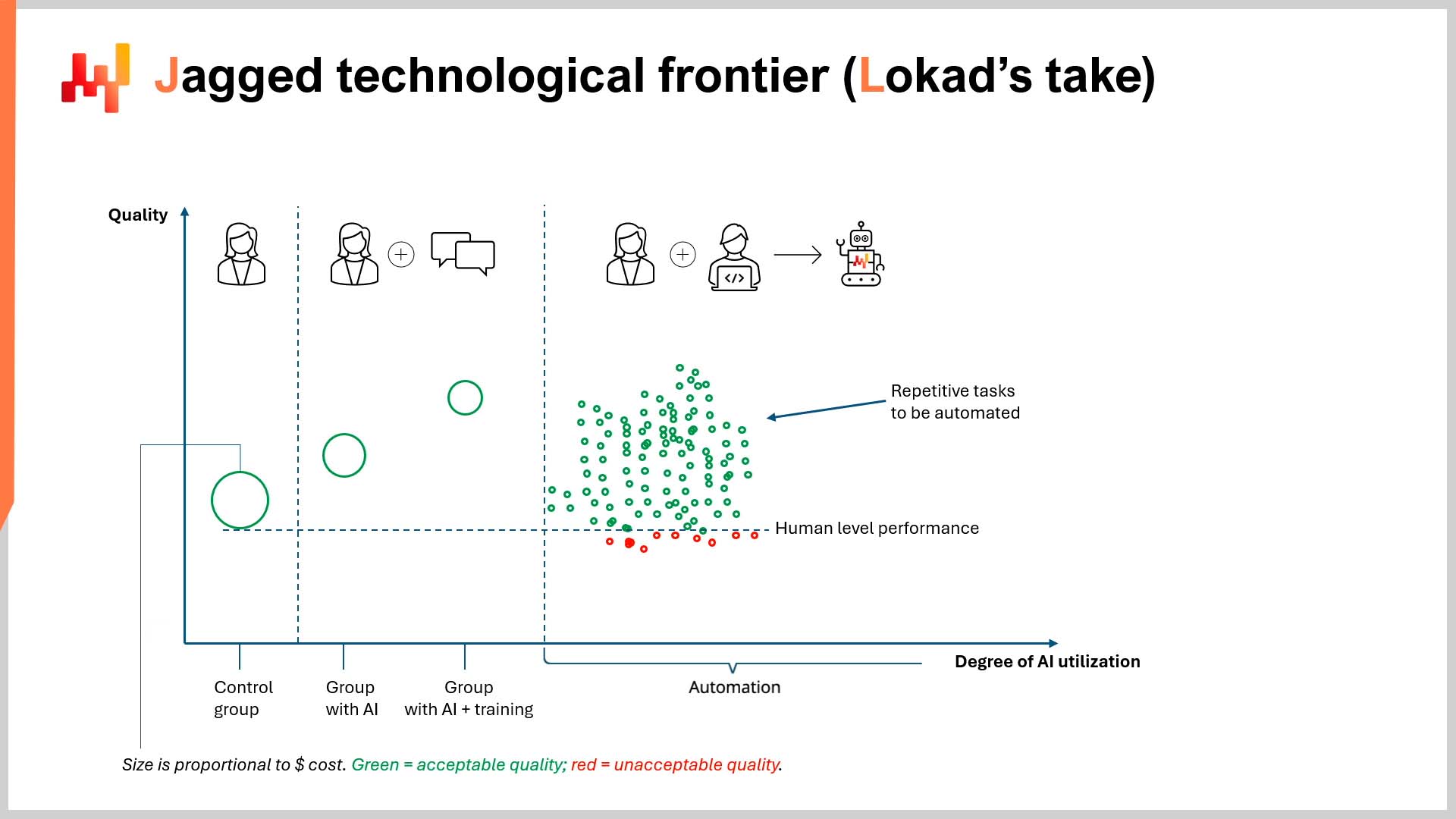
Context
On the far side of this “jagged technological frontier” (see illustration on p. 27 of paper), human expertise still outperforms AI (in this case, ChatGPT-4), particularly in tasks that merge quantitative and qualitative analysis (i.e., “outside the frontier” tasks).
This should not surprise anyone familiar with what large language models (LLMs) like ChatGPT are designed to do (spoiler: not maths). Also, when LLMs are treated as a “database of everything ” they produce plausible but sometimes inaccurate responses2. However, the paper still raises a few interesting points - though only accidentally.
To its credit, the paper is very readable, something often sorely lacking in academia. That said, one could raise a few concerns regarding potential conflicts of interest3 and its findings4, however it is the methodology and implicit economics of the paper that are of chief interest here.
Critique of Methodology
Regarding methodology, this point was already critiqued in the video, so I will be brief here. The research team did not explore the productivity gains generated through automation. Instead, the researchers selected groups of consultants (i.e., non-specialists in AI, computer science, and/or engineering) to use ChatGPT-4. The only exception to this was the control group, who worked using only their expertise. The ethics of how these groups were judged will be mentioned shortly.
There were no external software engineers or AI-experts in the experiment. No teams of developers adept at retrieval augmented generation (RAG), domain specific fine-tuning, or other techniques that utilize LLMs’ greatest strengths: being noise-resilient, universal-templating robots.
This impressive robot was not programmed to leverage the domain-specific knowledge stored in BCG’s extensive internal databases of past consulting initiatives. On the contrary, the experiment featured some consultants with a subscription to ChatGPT-4.
This orchestration naturally elicited varying degrees of output (in terms of quality), particularly regarding the tasks that required both quantitative and qualitative analysis. In other words: an experiment was designed to see just how badly non-experts fail to capitalize on a sophisticated piece of technology, and under unrealistically restrictive conditions.
As I concluded in the video, ignoring the possible productivity gains (and savings) generated through automation borders on scandal. This is especially true when one publishes under the name of a prestigious institute of learning. The paper’s findings also give, in my opinion, a very false sense of (job) security to students about to enter into life-altering debt in order to study at a top business school. This is also true for people who have already taken the plunge and incurred sizeable debts for diplomas in fields that may be on the cusp of full automation.
Critique of Economic Perspective
Despite the sizeable criticism above, by my lights, the implicit economics of the paper is much more fascinating. Simply put, Harvard Business School measured only $${quality}$$ of output, not $${cost}$$ of output5.
At no point (and please fact-check me) do the researchers measure the cost associated with the output of the consultants’ work. This is not a trivial point. The paper mentions the word “quality” 65 times in 58 pages (including the title of the paper). The word “cost” is mentioned…2 times…and only in the very last sentence of the paper. I reproduce that sentence here for context:
“Similarly to how the internet and web browsers dramatically reduced the marginal cost of information sharing, AI may also be lowering the costs associated with human thinking and reasoning, with potentially broad and transformative effects.” (p. 19)
Even when Harvard Business School finally acknowledged the concept of financial cost, it was not in terms of the reduced cost of generating high-quality work without the need for expensive business school graduates. This may seem obvious considering the paper’s title identifies “productivity” and “quality” as focal points of research, not to mention the fact that an expensive business school is hardly going to advertise their own potential looming uselessness.
That notwithstanding, I politely suggest that measuring $${productivity}$$ and $${quality}$$ of output without a robust financial perspective is practically meaningless, particularly in an academic paper from a business school. This is particularly egregious given the entire crux of AI is that it is a grand equalizer when it comes to fiscal firepower.
AI provides very high-quality results at very low costs, especially through automation. This $${quality/cost}$$ ratio is orders of magnitude beyond that of human workers6. Furthermore, it opens this door to anyone with a ChatGPT subscription and some knowledge of programming. This dramatically levels the playing field when it comes to competition between the big boys and the minnows.
Instead, for 58 pages, Harvard Business School assesses the “quality” of the BCG consultants’ work in isolation. How was this quality determined? By “human graders”…who work for BCG7. Putting to one side the manifest conflicts of interest already detailed, it is worth remarking on the false dichotomy presented by the paper, and how this influences its implicit economics. This false dichotomy runs something like this:
“AI is either better or worse than human expertise.”
Or perhaps a more charitable interpretation is:
“AI makes humans better or worse at their jobs.”
Either way, the metric underlying the false dichotomy in the paper is “quality”, which is subjectively measured and exists in an academic vacuum independent of other constraints, such as time, efficiency, and cost. A more sophisticated economics perspective would be something like:
What is the $${quality/cost}$$ ratio of human output compared to the $${quality/cost}$$ ratio of AI-automation?
Sophisticated readers will recognize this as an ROI (return on investment) argument. Your personal $${quality/cost}$$ ratio can be discovered by answering the following questions:
- How good was the output for a given task?
- How much did it cost?
- Was the quality worth the cost?
- How much would it cost to improve the quality, and would that improvement be financially worthwhile?
Harvard Business School spends 58 pages discussing the first question and never moves beyond it. This is a peculiar perspective for a business school, it must be said. In fact, one can draw an interesting parallel to supply chain. HBS’ blind pursuit of quality is remarkably similar to the isolated pursuit of forecast accuracy (i.e., trying to improve forecast accuracy without considering the ROI associated with that improvement)8.
Potential Implications
Economically speaking, the “jagged technological frontier” is not simply identifying what tasks LLMs perform better than humans. Rather, it is identifying your ideal $${quality/cost}$$ ratio when leveraging LLMs, and making intelligent, financially informed decisions. For savvy businesspeople, this will involve automation, not manual intervention (or at least very little of it).
For these businesspeople, perhaps a comparable level of quality is acceptable, so long as it is cost-efficient. “Acceptable” may mean the same as, or slightly better/worse quality than what a human expert can generate. In other words, paying 0.07% the price of a consultant for >90% of the quality might represent a very good tradeoff, despite not being exactly as good as the expensive expert’s output9.
The numbers will vary for each client, but what is demonstrably clear is that there is a tipping point beyond which “quality” ceases to be a concern in isolation and must be evaluated with respect to its financial cost. This is at least true for businesses intent on staying in business.
Perhaps you are of the opinion that hiring a team of Harvard Business School graduates, or consultants from BCG, represents an ideal $${quality/cost}$$ ratio, regardless of cheaper options like AI-automation. If so, I hope you live long and prosper10.
Alternatively, perhaps you think like me: quality is subjective; cost is not. My subjective appreciation of quality - certainly when it comes to business - is relative to its cost. Similar to service levels (or forecast accuracy) in supply chain, an extra 1% bump in quality (or accuracy) is likely not worth a 1,000% hike in cost. As such, when it comes to AI in business there is an economic tradeoff to be made between quality and cost. It is crucial not to lose sight of this, as it appears Harvard Business School has done.
In closing, if you are waiting for AI to surpass human quality before adopting it, so be it, but the rest of us are not going to wait with you.
-
Navigating the Jagged Technological Frontier: Field Experimental Evidence of the Effects of AI on Knowledge Worker Productivity and Quality, Dell’Acqua, Fabrizio and McFowland, Edward and Mollick, Ethan R. and Lifshitz-Assaf, Hila and Kellogg, Katherine and Rajendran, Saran and Krayer, Lisa and Candelon, François and Lakhani, Karim R., September 2023 ↩︎
-
In AI Pilots for Supply Chain, Joannes Vermorel compared this “Swiss Army Knife” approach to asking a very intelligent professor to recall the details of a paper they once studied. Off the top of their head, the professor will recall the gist, but they might not necessarily recall all the nuance unless you ask the right follow-up questions to help jog their memory. ↩︎
-
BCG helpfully advertises its ties with many of America’s top business schools. Feel free to investigate the presence BCG has on major US campuses. Alternatively, you can peruse this helpful downloadable Excel that simplifies the data. The table includes how many BCG consultants currently study MBAs at Harvard Business School (74). Readers may come to their own conclusions. ↩︎
-
A collaboration between a major business school and a major consulting firm demonstrated that expensive (and expensively trained) consultants are valuable assets… Forgive me for being less than shocked. If you are, though, I politely redirect you to the downloadable Excel in the previous footnote. ↩︎
-
And it most certainly did not measure quality divided by cost of output, as I will cover later. ↩︎
-
It is difficult to provide precise figures on this point but let us assume a yearly salary of over $200,000 (US) for a consultant from any given top firm. This figure is reasonable based on some cursory internet investigating. ChatGPT’s back-of-the-napkin calculations suggest a year’s worth of consulting work would cost about $145 (in terms of ChatGPT processing costs). Obviously, this is not terribly scientific, but even if the figure is off by three orders of magnitude, that is still almost 30% cheaper than the yearly salary of a single consultant. Consult ChatGPT’s reasoning here: https://chat.openai.com/share/d9beb4b9-2dd3-4ac2-9e95-2cd415c76431. (Credit to Alexey Tikhonov for providing the conversation log.) Admittedly, one must also consider the costs of constructing the templating robot itself, which might not be cheap, but even in combination with the $145 for ChatGPT would still be cheaper than a single consultant’s yearly wages and be exponentially more productive when deployed at scale. ↩︎
-
See pages 9 and 15 of the paper, in case you doubt what you just read. If this perhaps suggests a conflict of interest, I politely re-redirect your attention to the downloadable Excel in footnote 3. ↩︎
-
Lokad has addressed the value of forecast accuracy in detail before, so here is a very brief refresher: focus on the financial impact of your supply chain decisions, rather than measuring KPIs (such as accuracy) independent of the their financial implications (i.e., ROI). To put it another way, if a demand forecast is 10% more accurate but you make 20% less money as a result, it is fair to say the increased accuracy was not worth the increased cost. ↩︎
-
Approximately a year ago, Lokad began translating its website into six languages using LLMs. Prior to that, we had retained the services of professional translators. The ongoing cost to simply maintain the translations was about $15,000-30,000 (US) per year per language. While we are perfectly willing to concede that the professional translators provided (relatively) higher quality, the $${quality/cost}$$ ratio of using an LLM is far greater. In other words, the quality we can generate using an LLM is more than acceptable, and significantly cheaper than our previous arrangement. It is difficult to properly quantify the savings, but competently translating every single resource we have ever produced (and ever will) into six languages in a few minutes is exponentially cheaper and more time-efficient than the previous system. If you are a native French, German, Spanish, Russian, Italian, or Japanese speaker, feel free to translate this essay in the top-right corner of the page and test the quality for yourself. ↩︎
-
At least until OpenAI releases ChatGPT-5. ↩︎