Demand Forecasting through Envision - Workshop #4
The newest addition to our Envision workshop series1 (Workshop #4: Demand Forecasting) is now available for supply chain academics, educators, university students, and supply chain professionals. This workshop offers practical insights into how our Supply Chain Scientists (SCSs) use Lokad’s Quantitative Supply Chain perspective (and tools) to tackle demand forecasting for supply chains. This workshop is particularly important given how crucial demand forecasting is for producing optimized risk-adjusted inventory decisions.
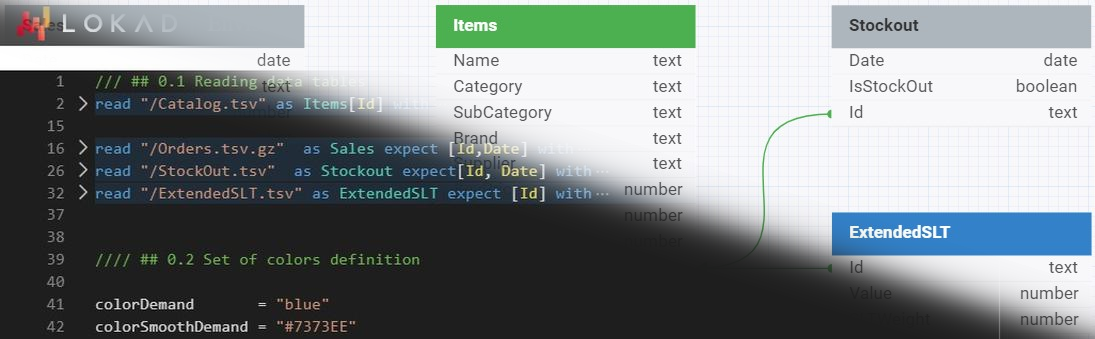
This workshop, like its predecessors, continues the form of a fictitious case study2 in the retail sector. It provides students with all the necessary information, guidance, and data to produce demand forecasts to anticipate future demand and drive timely inventory decisions that minimize financial risks for the retail company.
For educators, the workshop provides all the required resources for an engaging and instructive lesson in modern supply chain planning, including a dataset with a relational data schema, an interactive work environment, and a case study with embedded links to help students locate and use the relevant Envision code/functionality3.
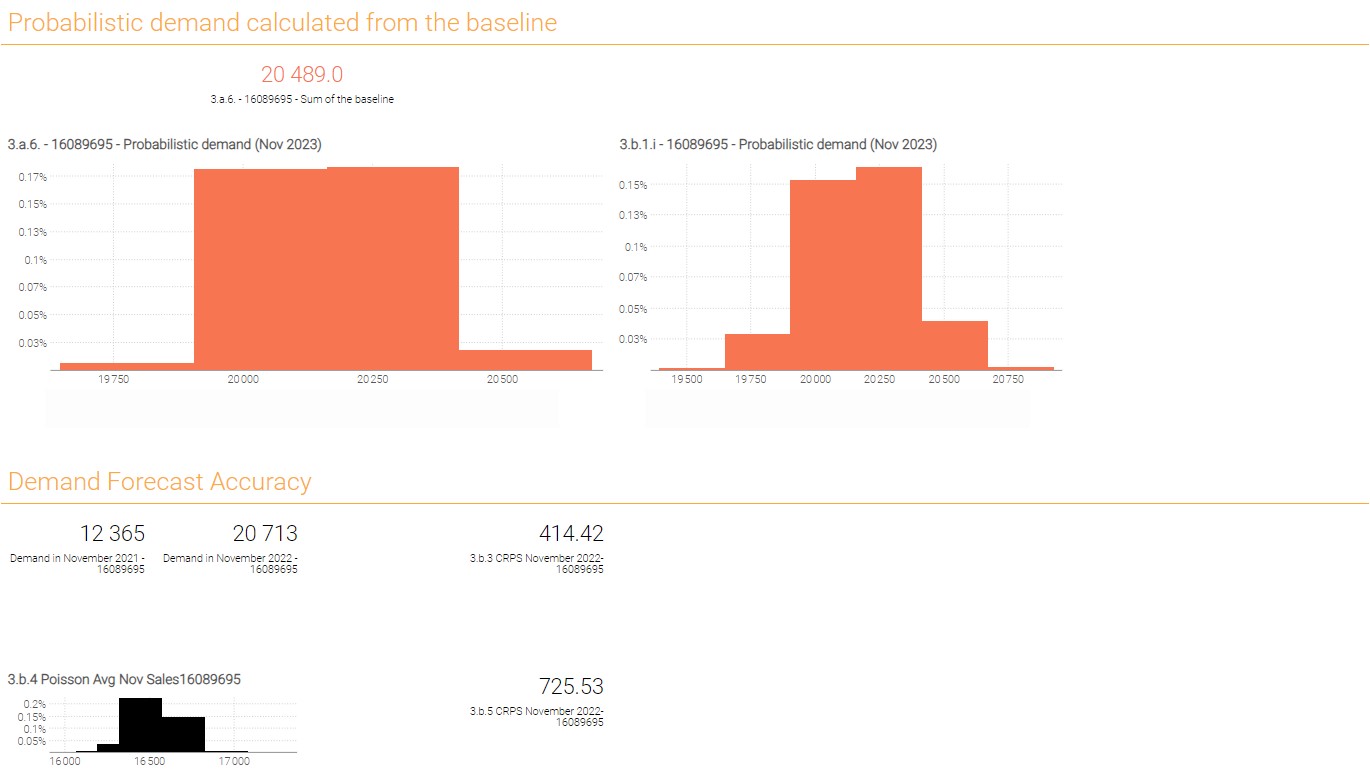
The primary goal of these workshops is to provide students structured guidance for analyzing modern supply chains. This process mimics the real-life business situations that Lokad’s Supply Chain Scientists (SCSs) generate recommendations for on a daily basis. It also mimics the progression of supply chain initiatives that Lokad implements with its clients. Each workshop is standalone, however there is value in studying them in the provided order as they all use data for the same fictitious company, with each workshop directing focus to different aspects of the company’s supply chain.
The workshop begins with an overview of the fictitious company, an introduction of key terms and definitions, and a thorough data description. This comprehensive description mimics a real-life Joint Procedure Manual (JMP) - the foundational document for every supply chain initiative with Lokad.
This new workshop should be especially interesting for the supply chain forecasters and demand planners as it provides an opportunity to learn more about probabilistic forecasting, which is the most comprehensive way of embracing future uncertainty4. It also shows the sorts of tools Lokad uses to completely automate the generation of forecasts on a daily basis. This automation requires careful designing of numerical recipes by the Supply Chain Scientist - a skill we believe every future supply chain practitioner will need.
In my opinion (shared by the professors who currently use our materials), the mainstream supply chain curriculum does not produce practitioners that are capable of effectively running even the supply chains of today - never mind the more complex and dynamic supply chains of tomorrow. I submit that the supply chain field of study deserves proper educational materials that directly address these challenges, and I hope our ongoing contribution will help to educate more aspiring Supply Chain Scientists.
In closing, I would like to extend my enormous gratitude once again to Paul Jan5 for his significant past and ongoing contributions to this project. Without his efforts, this workshop series would not have been as successful, nor as high-quality as I believe it is.
-
All published workshops as well as other practical exercises can be found in Envision Gallery section of Lokad’s public documentation. ↩︎
-
Supply Chain Personae is an alternative approach developed by Lokad to study real supply chains, and to address the flaws of traditional case studies. ↩︎
-
An answer sheet is available upon request. ↩︎
-
As depicted in the image, probabilistic forecasts do not typically look as fancy as Gaussian distributions. By design, optimizing the forecast for both computational efficiency and accuracy removes the eye-catching symmetry of a normal (Gaussian) distribution. Furthermore, supply chain data (and problems) are not normally distributed. Dive into the actual workshop materials to learn why. ↩︎
-
Paul Jan is, amongst other things, an Associate Professor at University of Toronto’s Rotman School of Management, where he teaches Operations and Supply Chain Management. He has more than 19 years of experience in the fields of education, manufacturing, and supply chain. ↩︎