A manufacturer's perspective
A few days ago, a prospect raised several sharp questions concerning the applicability of the Quantitative Supply Chain perspective to address the supply chain challenges as faced by many large manufacturing companies.
Let’s consider the case where there are a lot of items coming out of relatively few types of work cells/machines that can make any item in next week’s cycle, as long as there is the staffed capacity and raw materials. What does a company’s demand pattern and supply ability need to look like for probabilistic forecasting to be meaningfully superior to a good, standard APS like JDA or SAP APO? Wouldn’t aggregated forecasts, which are less erratic and hence a better fit for traditional forecasting and APS, be good enough for the problem at hand?
Probabilistic forecasting is not just about demand, but about embracing all aspects that remain irreducibly uncertain: demand but also lead times, returns, price changes, etc. The greater the uncertainty, the bigger the competitive advantage of any numerical approach that tackles uncertainty upfront instead of ignoring the problem altogether. Aggregating ‘classic’ forecasts is the numerical equivalent of sweeping the dirt under the rug. A monthly forecast might be more accurate - when measured in percentages - compared to a daily one; however, the extra accuracy is paid in extra market lag, as the statistical indicator is - by construction - spanning over a whole month.
Ignoring structural risks - such as losing a large client and all its ongoing stream of orders - is the recipe to generate an ongoing stream of dead inventory over time, as all clients will quit at some point - even if it’s to win them back a year later. At a more mundane level, ignoring lead time variability is causing inventory to be inefficiently allocated because, precisely, the stock is there to cover the lead time variance. Without a probabilistic forecast, the uncertainty is not even properly estimated. As a rule of thumb, varying lead times are never normally distributed.
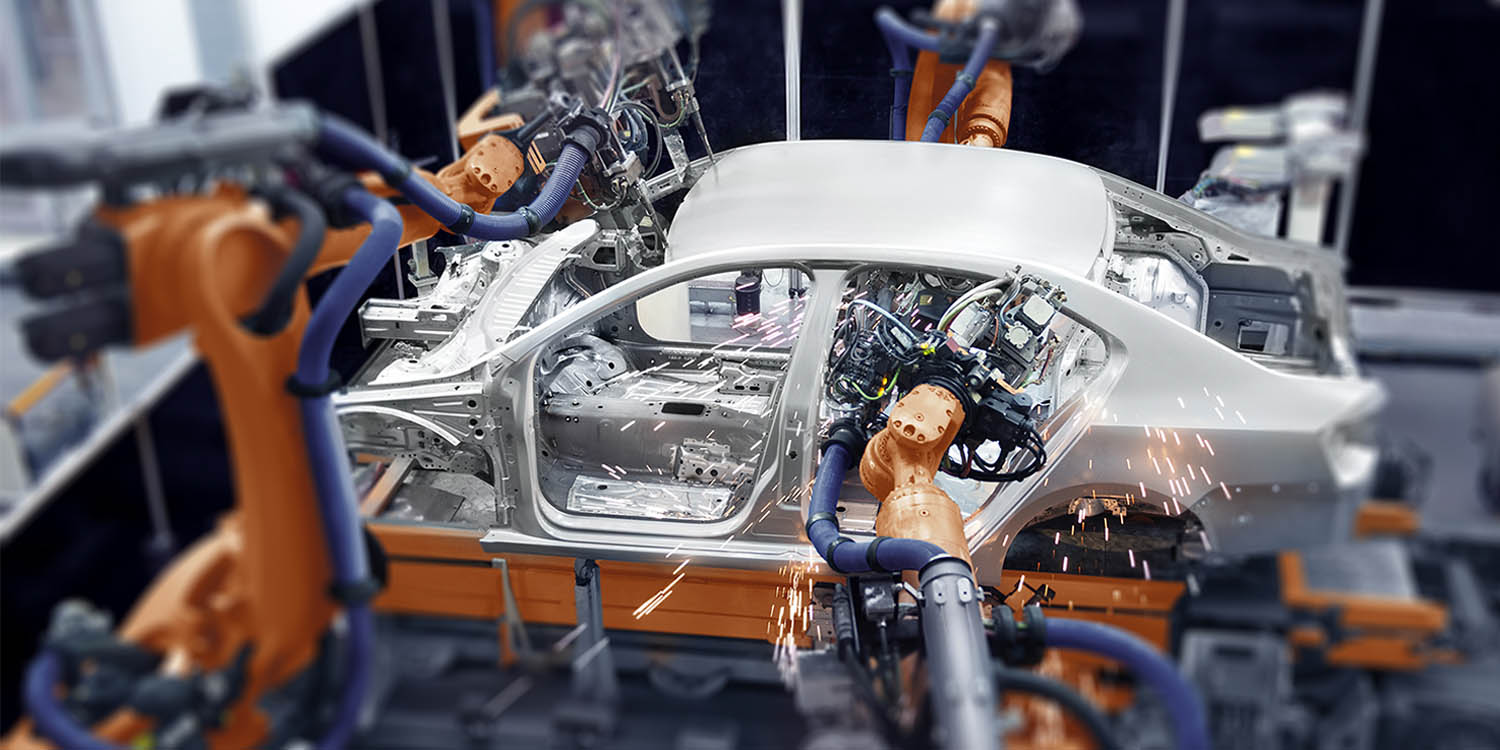
However, probabilistic forecasts are only numerical artifacts. At the end of the day, only decisions matter, their performance being measured in euros, not the intermediate numerical artifacts used to produce them - such as classic or probabilistic forecasts. The main weakness of APS is that they simply don’t optimize the supply chain from a financial perspective. Improving forecasting MAPE is vanity; only ROI matters. Probabilistic forecasts win, not because they are more accurate, but because they are vastly more convenient to turn into decisions optimized against arbitrary ROI criteria.
Thus, unless the supply chain financial stakes are inconsequential, APS are never “good enough” in my book, because the APS is not even making an effort at optimizing supply chain decisions from a financial perspective. In practice, supply chain teams end-up shouldering the entire responsibility of the financial performance through their Excel sheets; zero kudos is to be given to the APS for this.
Quantitative Supply Chain and DDMRP compete as respective numerical recipes for the operational horizon. However, doesn’t the Quantitative Supply Chain suffer similar challenges on supply constraints? Or do you model them explicitly? How do you drive the tactical horizon to project capacity tight spots sufficiently far out to give management a chance to fix them, e.g. by asking capacity?
Unlike DDMRP, Quantitative Supply Chain (QSC) does come with “packaged” numerical recipes. QSC is merely a principled approach to craft useful recipes, intended for straightforward production use, and to refine them over time. Gathering and nurturing numerical tools that are versatile enough to cope with the maddening diversity of supply chain constraints (e.g. MOQs, BOMs, cash flows, SLA penalties, etc) is a core concern for QSC.
At Lokad, we have been aggressively iterating from one tech generation to the next over more than a decade now. We have introduced two algebras to address this class of problems along with multiple non-linear solvers, the latest iteration at present date being differentiable programming. The whole point of those tools is to let a supply chain scientist - as pointed out - to explicitly model all those constraints. As the constraints themselves are diverse, it takes some programmatic expressiveness to even get a chance to adequately model those constraints.
Then, the financial perspective - one of the core principles of QSC - offers remarkable insights when it comes to supply chain constraints. In particular, it becomes possible to price the benefits associated with the lift of any given constraint. Indeed, the challenge is not so much asking to bring up the production capacity problem - but rather to justify the profitability of any investment to be made in this specific area.
In practice, there are (nearly) always multiple options that compete to address the same problem: maybe it’s possible to build up stock ahead of time instead of ramping up the production capacity, maybe it’s possible to increase the production batches to increase the throughput, maybe pricing should be increased when facing peak production capacity, etc. The QSC approach lends itself to ROI-based prioritization of all those options; the priority list being continuously refreshed along with the input data.
In practice, the only limit to looking far into the future is the statistical uncertainty that comes with it. Most “data-driven” investments - extra stock, extra capacity - are negatively impacted by market shifts, which tends to make them irrelevant. This problem impacts all quantitative methods - QSC and DDMRP alike - the only mitigation known to me being the explicit probabilistic forecasting.
Some of the demos from SAP on IBP show a lot of sexy stuff in being able to project and visualise the impact of a late shipment, as well as the ability to run a tactical horizon. Do you see Lokad playing there, thus eliminating the need for such tools? Or do you see APO/IBP as a relatively simple middle layer with these strengths used, but Lokad as a system of differentiation/innovation which yanks through the decisions to execute (purchase, production, transfer orders) and push them through APO/IBP?
Lokad is intended as an analytics layer that sits on top of a transactional layer, typically an ERP or a WMS. The intent is to generate finalized decisions that are already compliant with all the applicable constraints, removing the need for any further “smart” data processing. In this respect, Lokad occupies the same functional niche as SAP APO and SAP IBP.
As far as user experience is concerned, I believe that Lokad’s web dashboards are smooth and snappy as well. However, nowadays, producing nice-looking dashboards and what-if capabilities is relatively straightforward for software editors. Visualizing the impact of a late shipment is nice, but I am inclined to think that it’s not a very capitalistic way to leverage the time of the supply chain staff. Supply chain software all too often consumes an inordinate amount of manpower merely to keep running.
We take the opposite angle: every hour spent on Lokad should be invested in the betterment of the solution, whose execution is fully automated. Thus, going back to the example of a late shipment, I would observe that late shipment is merely the symptom of previous incorrect decisions: maybe reordering a bit too little a bit too late, maybe the choice of an unreliable supplier or of an unreliable transporter, maybe the incorrect prioritization of shipments between clients competing for the same stock, etc.
Focusing on the numerical recipe that generates all the mundane supply chain decisions is not very visually appealing - certainly not as much as what-if capabilities. Lokad can also deliver what-if capabilities, however, unless there is a clear path to turn those efforts into a stream of better decisions automatically generated by the solution, I don’t advise my teams to take such a path.
When considering supply chain configurations (open/closed production units and warehouses; which customers to allocate to which DC, etc)., the value of investing in agility and shorter lead times from a supplier or own manufacturing cell, i.e. Llamasoft supply chain design - typically driven off scenarios not possible to build from history: are these the types of questions one could use Lokad for?
In the late 90s, many experts had foreseen that the future of photography was digital and that argentic photography was doomed, but 20 years later, we are still decades away from having a machine learning technology capable of producing such high-level insights by merely “crunching” patent databases.
The Quantitative Supply Chain - and Lokad - is statistical at its core. When it comes to optimizing supply chain decisions that happen to be complete statistical outliers both by their magnitude and their frequency, e.g. deciding to open a new plant, the statistical perspective is at best weak and frequently misleading.
Considering the lead times, Lokad is much more suited to deciding whether air freight should be used - or not - for every single shipment, as opposed to deciding whether strategic suppliers should be moved back from Asia to North America.
As a rule of thumb, whenever a supply chain decision can be revisited on a daily basis, then it’s a good candidate for Lokad. Historical data doesn’t not have to offer a 1-to-1 match to the scenarios that are being envisioned. Exploring alternative affinities between customers and DCs is precisely the sort of problems that Envision has been designed to tackle.