Spare Parts Inventory Management with Quantile Technology
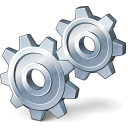
The management of spare and service parts is as strategically important as it is difficult. In a world where most equipment manufacturers and retailers are operating in fiercely competitive markets, a high service level to the existing customer base is a strategic priority for many players.
Not only does a high spare part availability help build a loyal base of customers, product/equipment companies have also discovered services as an often very profitable and recurring revenue stream that is typically more resilient to economic cycles than equipment sales.
However, managing a spare parts inventory efficiently still poses a huge challenge. Despite a forecasting and inventory planning technology industry that is several decades old, spare parts management has remained a difficult for a number of reasons:
- Large number of parts: Even smaller equipment manufacturers can easily be confronted with managing more than a hundred thousand spare parts.
- High service level requirement: Stock outs are often very costly, high to very high service levels are therefore paramount in many industries.
- Infrequent demand: The demand for spare parts is typically sparse and intermittent, meaning that only very low volumes are required occasionally.
Why standard forecasting technology performs poorly
Unfortunately, the combination of these factors makes standard inventory and forecasting technology ill-suited for spare parts planning. In classic forecasting and inventory planning theory, a forecast is produced by applying models such as moving average, linear regression and Holt Winters and a great deal of attention is given to the forecasting error, which is optimized by measuring MAPE or similar indicators. The transformation into a suggested stock level is done in a second step via classic safety stock analysis.
In the case of sparse time series (also called slow movers: low unit and infrequent sales), this methodology fails. The main issue with forecasting slow movers is that what we are essentially forecasting are zeros. This is intuitively obvious when looking at the demand history of a typical spare parts portfolio on a daily, weekly, or even monthly basis: By far the most frequent data point is zero, which can in some cases make up more than 50% of all recorded data points.
The challenge of forecasting slow movers: Good statistical performance and good inventory practice are not the same.
When applying classic forecasting theory to this type of data set, the best forecast for a slow moving product is by definition a zero. A ‘good’ forecast from a statistical point of view will return mostly zeros, which is optimal in terms of math, but not useful in terms of inventory optimization.
The classic method completely separates the forecast from replenishment. The problem is, the situation can hardly be improved with a “better” forecast. What actually matters in practice is the accuracy of the resulting inventory level (reorder point ), which is not measured nor optimized.
Changing the vision from Forecast Accuracy to Risk Management
When dealing with slow movers, we believe the right approach is not to approach the problem as a forecasting issue and to try to forecast demand (which is mostly zero). Much rather, the analysis should provide an answer to the question how much inventory is needed in order to insure the desired service level.The whole point of the analysis is not a more accurate demand forecasts, but a better risk analysis. We fundamentally change the vision here.
Determining and optimizing directly the Reorder Point
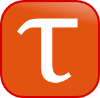
Quantile forecasts allow the forecasting of the optimal inventory that provides the desired inventory level directly: A bias is introduced on purpose from the start in order to alter the odds of over and under forecasting.
Benchmarks against classic forecasting technology in food, non-food, hardware, luxury and spare parts consistently show that quantile forecasts bring a performance improvement of over 25%, that is either more than 25% less inventory or 25% less stock outs.
In our opinion, by solving the problem of forecasting intermittent and sparse demand in spare parts management, quantile technology not only provides a strong performance increase, but also makes classic forecasts plain obsolete.
Whitepaper spare parts management available for download
Download the whitepaper Spare Parts Inventory Management with Quantile Technology for an in-depth discussion of the topic. Further whitepapers and resources on quantile forecasting and inventory management are available on our resources page.
Do you have comments, questions or experiences regarding spare parts management to share? Please participate in the comments below, your contribution is highly valuable to our team.
Reader Comments (1)
Great post on Inventory management technology. I would say from experience dealing with the forecast of risk management is a risk itself when you not doing it yourself and depending on others to get the job done.
Chris Toff (5 years ago)